Maximizing Data Center Networks With the Power of AI
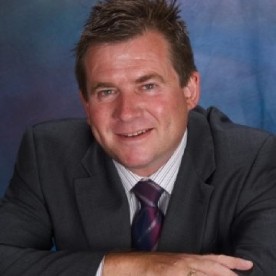
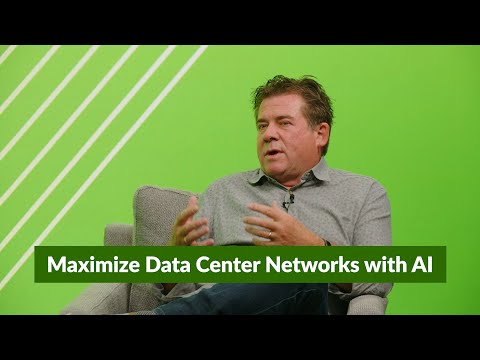
How to harness the power of AI to maximize data center performance.
Is your data center leveraging AI? See what experts at Juniper Networks have to say about how AI ops and infrastructure can improve overall data center performance in terms of speed, reliability, and efficiency.
For more, read, “Embracing the AI Revolution.”
You’ll learn
How AI makes data center troubleshooting more effective
About key AI use cases in the data center
How Juniper is positioned to partake in the next wave of AI investments by enterprises
Who is this for?
Host
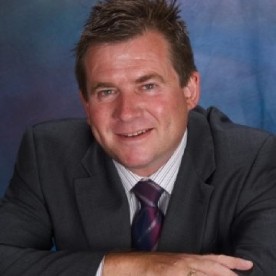
Guest speakers
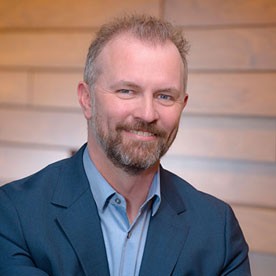
Experience More
Transcript
0:00 [Music]
0:05 hi everybody and welcome to our data
0:06 center video series uh my name is Dean
0:09 Sheffield and I'm joined by Scott sneden
0:11 um this is a a fireside chat Style video
0:14 series where we're going to try and
0:16 address some of the hottest topics uh
0:18 that we come across in our data center
0:21 experiences with our customers and
0:22 partners so we hope you find it
0:24 interesting um I think the first topic
0:26 uh we're going to address really has to
0:28 be Ai and ml mean it's it's wild it's
0:31 everywhere uh but what does it mean uh
0:34 for for data center operators people
0:35 building data centers people who are
0:37 putting their infrastructure in public
0:39 Cloud um there's there's a lot of a lot
0:41 of new buzzwords yeah a lot of stuff
0:43 going on so uh Scott what do you think
0:45 what's your first impressions well so at
0:47 Juniper anyway when we talk about AI
0:50 largely what we're talking about is mist
0:52 and and Marvis and the capabilities that
0:54 the team has built around making the
0:56 operational life easier making it easier
0:59 for Network people to run their networks
1:01 that's we we're leveraging AI really
1:03 heavily here to to lean into that to
1:05 deliver capabilities when we kind of
1:08 shift to Data Center and think about it
1:10 there's actually two topics so the first
1:12 is AI for operations and what we're
1:15 going to be doing with Marvis with
1:17 abstra with our intent-based networking
1:20 suite and bringing more AI capabilities
1:22 into that to just help operators do more
1:25 with less to do things faster to give
1:27 better insights and understanding so
1:29 that's a really
1:30 powerful tool that that a lot of that
1:33 exists today and a lot of that is
1:34 improving over time so so watch this
1:36 space the second topic around Ai and
1:40 data center is data center
1:42 infrastructure for AI workloads and that
1:44 is building very very large scale
1:48 high-speed switching capabilities and
1:51 fabrics to support these new AI
1:53 workloads um and that's also a really
1:55 exciting opportunity for us and there's
1:57 a lot going on there well let's break
1:59 that into to and let's let's have a a
2:01 chat about the the the AI Ops for the
2:04 data center operators I think some
2:06 people might be familiar with mist and
2:09 some of the challenges that mist helps
2:11 overcome specifically around
2:14 troubleshooting and finding out where
2:15 there's bottlenecks in the network in
2:17 the Wi-Fi network and in the actual you
2:19 know switching infrastructure yeah how
2:21 how does that sort of translate back to
2:23 the data center you think what what do
2:25 you think we can do there in the data
2:26 center I think it's what we're going to
2:28 see is is in machine capabilities to
2:32 make that troubleshooting more effective
2:34 we've already got an awful lot with
2:35 abster that's on the truck today a lot
2:37 of exciting things coming this year with
2:39 abster that make that even better with
2:41 visibility and monitoring and and uh um
2:45 tools to give you dashboards to really
2:47 understand and drill down to where
2:48 problems might exist and and try to
2:51 isolate and and troubleshoot things
2:53 before they become a major issue where
2:56 we take AI to the next level and and add
2:59 to that is to use some machine
3:01 capabilities to give you better Insight
3:03 better recommendations on where to go to
3:05 solve problems potentially even even
3:08 solving problems for you before they
3:10 happen I I think that's where we're
3:12 driving towards want to get to I I agree
3:14 I think
3:16 um the phrase meantime to innocence is
3:19 is a good one but also the faster you
3:21 can you know either isolate a problem
3:23 prove yourself innocent or at least help
3:26 remediate that problem yeah I I often
3:28 use the the phrasee the gift of time if
3:30 you can help people solve problems
3:33 faster that gives them more time to do
3:35 more you know productive things in the
3:36 data center what it's all about cool so
3:39 let's have a quick chat about the the
3:41 second thing you mentioned this this AI
3:43 infrastructure um I've done a little bit
3:46 of research and my my understanding is
3:49 that there's going to be uh obviously a
3:51 lot of data collection yeah uh we have
3:53 to gather all this data to then sort of
3:55 put into these training algorithms and
3:58 engines then at the back end of that
4:01 there has to be some other sort of
4:02 infrastructure that they call inference
4:05 infrastructure to try and you know bit
4:07 like chat GPT uh and B that that you
4:10 know once they've gathered the data and
4:12 trimmed the data down and then trained
4:14 it then we're going to see some new
4:16 things happening in the marketplace do
4:17 you want to have a a chat to me about
4:19 helping me understand the differences
4:21 between what that looks like from a
4:23 training of the data and then the
4:25 inference components to that yeah so the
4:28 um that sort of training of the data um
4:31 and and those those machine learning
4:33 clusters they're leveraging GPU type
4:36 CPUs they're um extremely high bandwidth
4:40 capable machines they operate in a
4:43 cluster so you've got multiple machines
4:44 communicating with each other those gpus
4:47 are extremely expensive um if you're
4:50 going to get your return on investment
4:52 from buying those machines and using
4:53 them the network that supports them has
4:55 to be non-blocking fully available um
5:00 very tightly managed for bandwidth and
5:02 capability so that it's always up and
5:05 these processes can run really quickly a
5:07 lot of that Market's in fiban today but
5:09 we do believe that it's going to move to
5:11 Ethernet there are a lot of reasons for
5:13 that around standards and multivendor
5:15 capabilities and we're investing heavily
5:18 in capturing that market so there's a
5:20 lot happening with with uh Juniper
5:22 around that and then as we look Beyond
5:26 just that piece of the cluster there's a
5:28 lot of of infrastructure to support
5:30 these workloads that looks like ethernet
5:32 that looks like a data center Fabric and
5:35 so what we have on the truck today
5:37 around high-speed switching um highly
5:40 available architectures automated abstra
5:43 driven intent-based Network
5:45 infrastructure to support any kind of
5:47 workload is very applicable to the
5:50 inference servers the sort of front-end
5:52 servers to these AIML machine you know
5:55 clusters so um yeah as far as building
5:58 infrastructure for this there's a lot of
6:01 stuff on the works to kind of get high
6:03 density high radics they call it
6:05 switching platforms um to support those
6:09 those backend needs and then on the
6:11 front end um what we have today is very
6:14 applicable and we're excited about it
6:16 excellent
6:17 so I'm thinking about use Cas use cases
6:20 now I I I did read something I think it
6:22 was like two weeks ago about some
6:25 Enterprises I think AT&T made an
6:27 announcement workday made an
6:28 announcement about
6:30 using AI type chat Bots and leveraging
6:34 training models
6:35 Etc I think there's some applicability
6:38 for you know some financial institutions
6:40 and some you know Healthcare
6:42 institutions where there's going to be
6:44 some sensitive data that's going to have
6:46 to be put into a a training model that
6:49 may be on Prem and not leveraging public
6:52 you know infrastructure that's out there
6:54 um any interesting use cases you can
6:56 think of around that well I I just like
6:59 we've seen with General Cloud workloads
7:01 there's a lot of sort of if not
7:03 repatriation at least reconsidering
7:06 where the best place is for a workload
7:08 and that carries forward with these AI
7:10 workloads as well um economically maybe
7:12 it makes sense to leverage a public
7:15 available learning cluster to get
7:17 started especially because they have
7:18 expensive they are very expensive but if
7:21 that's your business's crown jewels I
7:23 think we're going to see a lot of
7:24 investment within Enterprise to build
7:26 that stuff inh housee um so maybe Hybrid
7:29 models maybe there are you know leasing
7:33 for time on some almost bare metal as a
7:36 service type things but I I think we're
7:39 going to see a lot of Enterprises
7:40 building their own AIML capabilities in
7:43 I think it's very exciting in fact I've
7:44 seen some of the the forecast for the
7:46 addressable Market that that's going to
7:48 be out there and it uh it's people sting
7:52 billions of dollars of of of Investments
7:54 going into this space so extremely
7:57 exciting uh you know I think we're
7:59 extremely well positioned to partake in
8:01 this next boom and uh you know let's see
8:04 what we can do yep great excellent
8:06 thanks very much for for tuning in uh to
8:09 our first episode um we we'll be back
8:11 with another one very shortly appreciate
8:13 your time cheers thank
8:15 [Music]
8:21 you