Juniper’s Bob Friday: Preparing for AI in Your Business


AI is accelerating. Is your organization ready for it?
Tune into this eWeek interview, starring Juniper’s own leading AI expert Bob Friday, who shares the steps your business needs to take to successfully deploy AI, including the need for quality data that can be processed in real time. Listen as Bob shares his thoughts on the rollout of AI and how AI will change the industry as it expands.
You’ll learn
One reason why Bob left Cisco to start Mist
How AI is the next step in the evolution of automation
What Bob thinks he’ll see happen with AI before he retires
Who is this for?
Host

Guest speakers
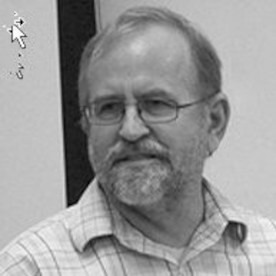
Transcript
0:01 [Music]
0:03 hi i'm james mcguire
0:05 and today we're talking about getting
0:07 your organization ready for artificial
0:09 intelligence
0:10 which of course includes making sure
0:12 your ai system is fed
0:13 enough data enough quality data to
0:16 discuss that i'm joined by a real expert
0:17 in the field
0:18 with me is bob friday cto of juniper's
0:21 ai driven enterprise business
0:24 bob very good to have you with us today
0:25 yeah i know james thank you for having
0:27 me here
0:27 you know ai's you know a topic dear to
0:29 my heart so it's really great to be here
0:31 with you today
0:32 great you know there's a there's a
0:34 really interesting uh report about
0:36 the state of artificial intelligence it
0:38 seems like there's a there's a bit of a
0:39 gap between
0:40 some of some of the hopes and some of
0:41 the optimism for ai and some of the
0:43 reality of how it's actually getting
0:44 deployed
0:46 especially a report i think it's either
0:48 sponsored or put together by juniper
0:50 uh it'd be nice for me to do a little
0:51 screen share and show the stats for
0:53 which i think it's
0:54 let me do this kind of advanced
0:56 technology the
0:57 zoom screen sharing uh here we go let's
1:00 do share
1:02 yeah and if i if i look at this it's
1:04 interesting so we look at the figures
1:07 the survey found that 95 of 700
1:10 respondents
1:10 believed that their organizations would
1:12 benefit from embedding ai into their
1:14 daily
1:14 operations so you know everyone really
1:16 wants to do it on the other hand
1:18 this compares with only six percent of
1:21 c-level leaders
1:22 who have reported adoption of ai power
1:24 solutions across their organization so
1:26 there's a real disconnect between uh
1:29 you know the optimism the hope for ai
1:31 and and what people want it to do or
1:33 think it can do
1:34 and the reality of how it's deployed
1:36 what what's going on there
1:38 to create that gap yeah you know james
1:40 when you look at it
1:41 you know i used to be the mobility cto
1:43 at the at cisco right you know
1:45 it would really start you know what i
1:47 heard there is really from customers
1:49 there's a couple things those customers
1:50 really were looking to do one is they
1:52 really wanted to get the
1:54 they wanted to basically get their
1:55 systems to stop crashing uh but they
1:57 really wanted
1:58 end-to-end visibility you know from the
2:00 client to the
2:01 to the cloud and that's really when ai
2:04 ops started to become more critical
2:05 and what i really noticed was you know
2:08 and that's one reason i
2:09 i left cisco to start mist right is
2:11 because what was really happening was to
2:13 build an ai
2:14 ops solution you really needed really to
2:16 have kind of a real-time pipeline
2:19 to deal with the data right it was
2:20 basically an architectural change what
2:23 was happening
2:24 you know from what i did 20 years ago at
2:26 airspace we built systems that were
2:27 helping enterprises manage
2:29 network elements you know really the
2:31 paradigm shift is really now helping
2:33 enterprises
2:34 really manage the end-to-end user
2:36 experience and that really requires you
2:38 to change two things
2:40 technically it requires something in the
2:42 cloud that can actually process this
2:44 data real time
2:45 right interesting the other interesting
2:47 twist i found in this
2:48 is really it requires almost an
2:50 organizational change
2:52 right culture a cultural change i'm
2:53 assuming a cultural change in both the
2:55 customer and in the vendors right so so
2:57 i missed
2:58 what we ended up doing was i ended up
3:00 really tying my
3:01 data science team up to my customer
3:04 support team
3:05 you know because one of the challenges
3:07 is you know you know we have data
3:08 scientists who
3:09 you know they understand the math and
3:11 the science behind the
3:12 ml algorithms but they're not
3:14 necessarily network domain experts
3:16 so you look at the challenges here you
3:18 know you got the vendors who've got to
3:20 change their culture
3:21 you know we got to move to architectures
3:23 that can actually do
3:24 aiml and you got to organizationally
3:27 change on the vendor side
3:28 and then on the customer side you know
3:30 the customers have to basically start
3:33 changing their it teams right you know
3:35 they're going through a bunch of changes
3:36 on
3:37 the it side you know you look at it
3:39 teams you know we were asking it teams
3:41 to do a
3:43 almost an exponential change right how
3:45 they operate their networks
3:47 yeah because because of the level of
3:49 data in other words well the complexity
3:51 right i mean you look at what's
3:52 happening on that site you know their
3:53 networks are getting more complex
3:55 and we're asking it guys to basically go
3:57 from being cli
3:59 programmers to being python programmers
4:01 right you know so they're already making
4:03 that shift to becoming
4:05 python programmers and now we're asking
4:07 them to become
4:08 ai data scientist experts right so
4:10 that's a lot of pressure on our
4:12 you know our customers right now and
4:13 getting their it team skill sets
4:15 up to the next you know next stage you
4:18 may you mentioned ai
4:19 ops so this is really is is that a key
4:21 area of yours in particular especially
4:22 in terms of you know dealing with the
4:24 complexity and
4:25 data and the data in the it operations
4:27 is are you are you big at ai ops in
4:29 particular
4:30 yeah that i mean that is what we do here
4:32 right now and that's really
4:33 that's really the change right ai ops is
4:35 really that paradigm shift from
4:36 managing network elements to managing
4:39 client to cloud right
4:40 you know i really don't care what's
4:42 between the client the cloud whether
4:43 it's
4:44 juniper equipment or someone else's
4:45 equipment my mission in life is really
4:47 to help those
4:48 help them figure out why their devices
4:50 and customers are having problems
4:51 internet poor internet connectivity
4:55 let's talk about the the ai uh ai ops
4:58 market for a second
4:59 how do you think is it is it close to
5:00 mainstream or is it still
5:02 in its infancy in terms of adoption what
5:04 would you say
5:05 you know i think right now we're really
5:07 in the early early days of aiops
5:09 adoption right
5:10 you know you know you look at enterprise
5:12 businesses you know like we mentioned
5:14 before
5:14 i mean culturally they're trying to get
5:16 their it teams you know with the right
5:17 skill sets to really embrace ai
5:20 and they're really really trying to
5:21 understand you know what is what's the
5:23 difference between ai
5:25 and automation right and from my
5:27 perspective
5:28 you know ai is really just the the next
5:30 step in the evolution
5:32 of automation right what we're really
5:34 doing
5:35 is we're really building things
5:37 solutions on par
5:39 they can do things on par with humans
5:41 now right it's kind of like a
5:42 self-driving car
5:43 diagnosing cancers you know and if you
5:45 look really one of the inspirations that
5:47 missed right you know one of the reasons
5:48 i started this was
5:50 you know when i saw watson play jeopardy
5:52 i don't know
5:53 right yeah it was it was a big deal when
5:56 yeah absolutely
5:57 you know that that's when i kind of
5:58 realized hey you know if they can build
6:00 something that can play jeopardy
6:02 right we should be able to build
6:04 something that can basically answer
6:06 questions
6:06 and manage networks on par with
6:10 network domain experts right like you
6:12 know the technology is real
6:14 we've gone from marketing hype to that
6:15 there is real technology here that we
6:17 can actually build something that can do
6:18 something on par with a human
6:21 well it's true but in terms of the the
6:22 you know the weaning of jeopardy that's
6:24 that's spitting back out information
6:28 uh which of course is going to be and
6:29 handling information processing
6:31 information which is going to be one of
6:32 the cores of aiops
6:34 but it's also i think about those i.t
6:36 experts
6:37 they're making nuanced decisions uh
6:40 they're they're
6:41 they're actually having to forecast
6:43 things and it says we need
6:45 we need aiops to do more than just spit
6:47 out the information we need we need
6:48 prediction and forecasting
6:49 we also we'll almost need the system to
6:51 be giving us a little bit of advice am i
6:53 am i correct for that
6:55 well yeah and that's what we're starting
6:56 to see right i mean we're starting to
6:58 see
6:58 systems now that can actually predict
7:02 hardware failures or find rma failures
7:04 inside the network right
7:05 you know so the days of you know where
7:07 you used to have to argue with your
7:09 vendor about you know whether or not
7:10 you're having a hardware software
7:11 problem
7:12 those days are going away because these
7:14 more intelligent systems they
7:16 know whether or not it's a hardware
7:17 problem software problem you know so
7:19 my transition from what i did 20 years
7:21 ago at airspace you know where
7:23 basically a customer would have to you
7:24 know send all bunch of information to
7:26 convince me it was a hardware problem
7:28 we are now we're sending rmas out right
7:30 you know customers don't have to wait
7:31 for me we can basically tell them yes we
7:33 know that you have a hardware problem
7:35 please send it back or other use cases
7:38 like bad cables
7:39 you know we've gotten down the point now
7:41 we can actually find these needle in the
7:42 haystack
7:43 bad cable problems where we're 90
7:47 or better accurate where i t departments
7:49 now i can say hey i trust you
7:50 you know don't don't bother me or if you
7:53 find a bad cable issues a support ticket
7:55 and and move on so those are kind of
7:57 cases where
7:58 we're starting to see ai and ml really
8:01 take on the this next level of
8:03 automation right
8:04 helping helping offload i t task onto
8:07 these ai solutions
8:09 so so what what is the name of the ai
8:10 ops solutions that actually can find a
8:13 bad cable
8:14 amid all those cables in in the data
8:16 center is there a specific solution that
8:17 does that
8:18 well i mean so so juniper we call it
8:20 marvis uh exactly
8:22 if you dig under the hood and actually
8:24 look at it you'll see something called
8:25 logistic regression
8:26 so that's kind of the actual ml
8:27 algorithm that's down
8:29 in the uh under the hood there is
8:31 actually making the determination
8:32 whether or not you have a bad cable or
8:33 not
8:35 interesting let's talk about uh the data
8:38 challenges what what data challenges
8:40 do organizations need to address in
8:43 order to quickly inform ai systems
8:45 because of course they need to be fed
8:46 the right the right data how do
8:49 companies do that
8:50 yeah and to be honest i mean like i said
8:52 back when we started mist right
8:54 i mean one of the questions when we
8:55 started this was you know should we
8:57 actually build an access point
8:59 or not right you know and when i made
9:01 the decision
9:02 back then was i wanted to build the
9:04 access point not because i thought the
9:05 market needed another
9:06 wireless access point it's because i
9:09 really want to make sure i can get the
9:10 data i need to answer a question
9:13 when i look at most enterprises right
9:15 now the mistake they're making is
9:18 they tend to focus all their energy on
9:20 trying to build big data lakes
9:22 data warehouses without really starting
9:24 with the question
9:25 right and so usually the advice i give
9:28 people is like hey if you're going to
9:29 work on an ai
9:30 ops ai type of problem you know you
9:33 really start with the question you're
9:34 trying to answer
9:35 you know in our case it was we're trying
9:37 to answer the question of why are you
9:38 having poor internet connectivity
9:41 and so that really starts with making
9:42 sure you get the data from the edge of
9:44 the network you know if you
9:45 start with that then it starts to drive
9:47 to make sure you get the data you need
9:49 to answer the question
9:50 you're trying to answer so the idea is
9:52 make make sure you know your question
9:54 you're sort of
9:55 guiding leading question as opposed to
9:56 merely buying technology
9:59 right or trying to pile data in a day
10:01 like right you know you see a lot of
10:03 enterprises so the first thing they try
10:04 to do is they try to take
10:05 every piece of data they have and start
10:06 you know you know sticking into splunk
10:09 or sticking to some big data warehouse
10:10 and then
10:11 then they find out they're not using 95
10:14 of the data never gets used for much of
10:16 anything right
10:18 it is amazing that the small amount of
10:21 data that is actually mined for
10:22 competitive advantage i mean that's i
10:23 think it's sort of the story of our of
10:25 our time is that
10:26 we have an ocean of data but we're using
10:28 just a small portion of it to really
10:30 navigate right and i'm going to add you
10:34 know if you look at the question right
10:36 you know as we've got you know what
10:38 we're doing now is we're starting to
10:39 expand
10:40 across different data sources right you
10:41 know when we started it was basically
10:43 getting data from access points to
10:46 answer the connectivity question
10:47 now we're getting data from the clients
10:49 you know their visibility of the network
10:51 we're getting data from the switches
10:52 and the routers you know and as you
10:54 start to get more data
10:56 it lets you answer questions more
10:57 questions like when we get data from the
10:59 sd-wan
11:00 router it starts to let us answer
11:01 questions about applications and
11:03 services
11:04 and we start to be able to answer
11:05 questions with more granularity
11:07 and then you can start doing proactively
11:09 fix it once you have data from the
11:10 switches
11:11 you know if we see a misconfigured vlan
11:14 we can actually practically correct that
11:15 without
11:16 bothering the i.t teams right right
11:20 you know we we talked about this a
11:21 little bit beforehand the idea of a
11:23 common telemetry
11:24 standard so what why does there need to
11:26 be a common telemetry
11:28 standard to make obtaining data for ai
11:29 easier why is that important in your
11:31 view
11:32 well i think it's important i think
11:33 that's one friction point we see in the
11:35 adoption of ai
11:37 is are we really going to get to a
11:38 common language where every vendor uses
11:41 the same you know
11:42 format for time um i think that's
11:45 probably a little hard
11:46 i think what is probably required in the
11:48 industry is if we get everyone to kind
11:49 of agree to how to store data away
11:52 you know when we look at you know what
11:53 question or what problem we're trying to
11:55 save
11:55 you know you may have to get data from
11:57 your access vendor right and you may
11:59 have to get data from your
12:00 lan router vendor and you may have to
12:02 get some data from your application
12:04 vendor
12:04 you're really to answer an end-to-end
12:06 type of question
12:08 so i think what's more important in the
12:09 industry right now is you know can we at
12:11 least get people to agree
12:12 on how they're going to store the data
12:14 way in these cloud solutions
12:16 because if you look where we're headed
12:17 right you know the industry is moving to
12:19 kind of
12:20 cloud first direction right we're almost
12:23 you know all the data you need is being
12:25 stored away you know
12:26 your different vendors are throwing this
12:28 data for you on your behalf
12:29 in different clouds so it's more
12:31 important about getting the data stored
12:32 away in some sort of descriptive format
12:34 that ai and bi can actually access and
12:37 understand how the data is being stored
12:40 is is it realistic to think that there
12:42 would be an industry standard in terms
12:43 of that or is it
12:44 is it inevitable or is it is it a long
12:46 shot in europe
12:48 i think it's an inevitable i think we're
12:49 in the early days of ai and so i think
12:51 people are just now fully
12:52 starting to appreciate the fact and i
12:54 think a lot of the aai
12:57 efforts are being kind of focused on
12:58 very narrow efforts where the data is
13:01 you know coming from one source or one
13:03 vendor you know so i think as you know
13:05 as ai expands especially iops and
13:07 network and expands
13:08 across networking we'll start to see the
13:10 industry move to more
13:12 industry standards like uh you know on
13:14 the switch side we moved to open config
13:16 right that was kind of a standard
13:18 effort to get the switches managed i
13:20 think so i do think start to see the
13:21 industry move to kind of more
13:23 standard ways of getting telemetry data
13:26 from different devices
13:27 especially on streaming right you know
13:29 pulling and pushing
13:30 you know streaming data yeah streaming
13:33 data you know you look at ai
13:35 uh you know in the past you know 20
13:37 years ago was all snmp right we were
13:39 basically pulling all these devices to
13:41 get stats
13:41 and information out of our devices as we
13:44 move into more of a real
13:45 time ai environment it's more about i
13:48 want things to be streamed to me in real
13:50 time i don't want to go pull things i
13:52 want to have telemetry come at me
13:54 so it's probably more important that we
13:55 get that standardized
13:57 as opposed to actually trying to
13:59 describe each little
14:00 field in the in the in the schema right
14:04 let's let's look to the future for a
14:05 second i i think specifically the future
14:07 not of overall ai necessarily but first
14:10 the future of ai apps in particular
14:13 you know when you look at the definition
14:14 of ai apps it's artificial intelligence
14:16 for it operations and that's really the
14:18 commonly accepted one i think gartner
14:19 made
14:20 that up in 2017. um
14:23 but i think about this obviously going
14:25 to be an ai platform that runs
14:28 the entire enterprise from sales to it
14:32 to you know hr and it's all going to be
14:34 one platform so really the idea of ai
14:36 ops which
14:37 you know our ai for it operations
14:40 is going to be consumed into a larger ai
14:44 framework true false or what's your view
14:47 on that
14:48 well i mean i think there's a lot of
14:49 concepts here you know one of them is
14:51 this ai for me personally like i said ai
14:54 is really about
14:55 trying to do something on par with a
14:56 human you know whether it's
14:58 driving cars or detecting cancer and
15:00 medically
15:01 for networking it's more about can we
15:03 build something that does something on
15:04 par with network domain experts
15:06 you know and i think the future is you
15:08 know and my common things i think
15:10 you and i probably grew up i don't know
15:11 if you remember watching star trek when
15:13 you were a kid right i do
15:14 yep definitely you know in every almost
15:16 every technology that was in star trek
15:19 30 40 years ago has now separated
15:22 except for the transporter right the
15:23 transporters i'm not sure we're gonna
15:25 live to see that
15:26 yeah computer remember the computers
15:28 like computer tell me
15:29 oh definitely yeah no it's and it's here
15:31 yeah yeah so i think we are gonna see
15:33 that in our future where
15:35 you know network it teams are gonna have
15:37 an ai assistant on their team
15:40 right that's going to make it easier for
15:42 them to diagnose
15:43 and run and maintain these networks just
15:46 like we saw on star trek right
15:47 and this is what i call the transition
15:49 you know to conversational interfaces
15:52 you know you can you kind of remember
15:54 the industry you know
15:55 when you're younger it's all about cli's
15:57 right we're basically programming and
15:58 maintaining all these
15:59 switches and routers you know i mean cli
16:02 jockeys you know
16:03 and then we kind of move to the
16:05 dashboard the gui dashboards for running
16:07 and manage our networks
16:08 right i think we're now in that
16:09 transition to conversational interfaces
16:12 are just a much easier way for network i
16:14 t
16:15 teams to actually manage and extract
16:17 data
16:18 and visibility on other networks so i
16:20 think that's kind of the future of
16:22 what i call ai in networking is the
16:24 virtual system think star track then
16:25 computer
16:26 you know you're going to start to see
16:28 virtual systems become a key element
16:30 in most of these network i.t teams in
16:31 the future so that means that
16:34 that nlp will be really at the center of
16:37 it all so that the i.t
16:38 expert can speak to his or her machine
16:40 yeah nlp is a great example right now of
16:42 these conversations and that that's an
16:44 area that's changing as we speak
16:46 right now i mean i can tell you there
16:48 are things that we can do now with the
16:49 conversational interfaces that we
16:50 couldn't do two years ago right
16:52 you know so that technology is evolving
16:54 you know it's still evolving right now
16:56 right so so what about the future of ai
17:00 ops
17:00 specifically if you look at this you
17:03 know
17:04 they're expecting something like 15 to
17:05 20 percent annual growth in in revenues
17:08 from about 17 billion now at about 40
17:10 billion in the year 2026
17:12 based on i think at idc numbers what do
17:15 you see
17:16 we the future of ai up specifically
17:20 going forward
17:21 yeah so specifically you know in in the
17:23 short term i think right now we're gonna
17:25 see you know as i said data you know
17:29 data is gonna be the i think it's gonna
17:30 happen in phases and data is probably
17:32 the first phase
17:34 you know where enterprises are working
17:35 on making sure they can get the data
17:37 that they need to solve some aips
17:39 problem right
17:40 and as we success earlier right so you
17:43 know i make a barrel of wine every year
17:45 and you know names red wine or white
17:47 wine red wine
17:48 yeah okay yeah so but uh you know same
17:52 saying right
17:53 great wine starts with great grapes
17:54 great the great data
17:56 and so that's probably the first phase
17:58 of the journey that most people are
17:59 going to get on is get that down
18:01 and then you gotta really get that data
18:02 into a framework that you can actually
18:04 start deploying
18:05 ml to and that's kind of real-time
18:07 pipeline
18:08 you know so that's kind of the future i
18:10 see the journey
18:12 uh onto the adoption of ai ops into
18:14 ultimately
18:15 you know before we before i retire i
18:18 suspect we're going to start to see
18:20 these virtual ai assistants become a
18:22 mainstream of uh
18:23 the i.t teams of the future yeah it
18:26 doesn't seem like that
18:27 particular one is too far away actually
18:29 that could be in the next couple two
18:31 three years i would think
18:32 yeah before before i retire right
18:35 okay good uh bob i think you've said it
18:38 there's a lot more to say but i think
18:39 that's
18:39 that's we're good for today i greatly
18:42 appreciate you sharing expertise with us
18:44 thank you very much yeah great to be
18:46 here james