IBM: The Role of Edge Computing in Digital Transformation
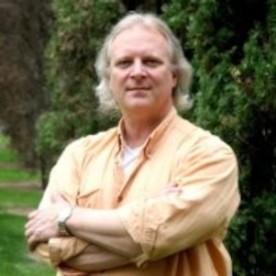
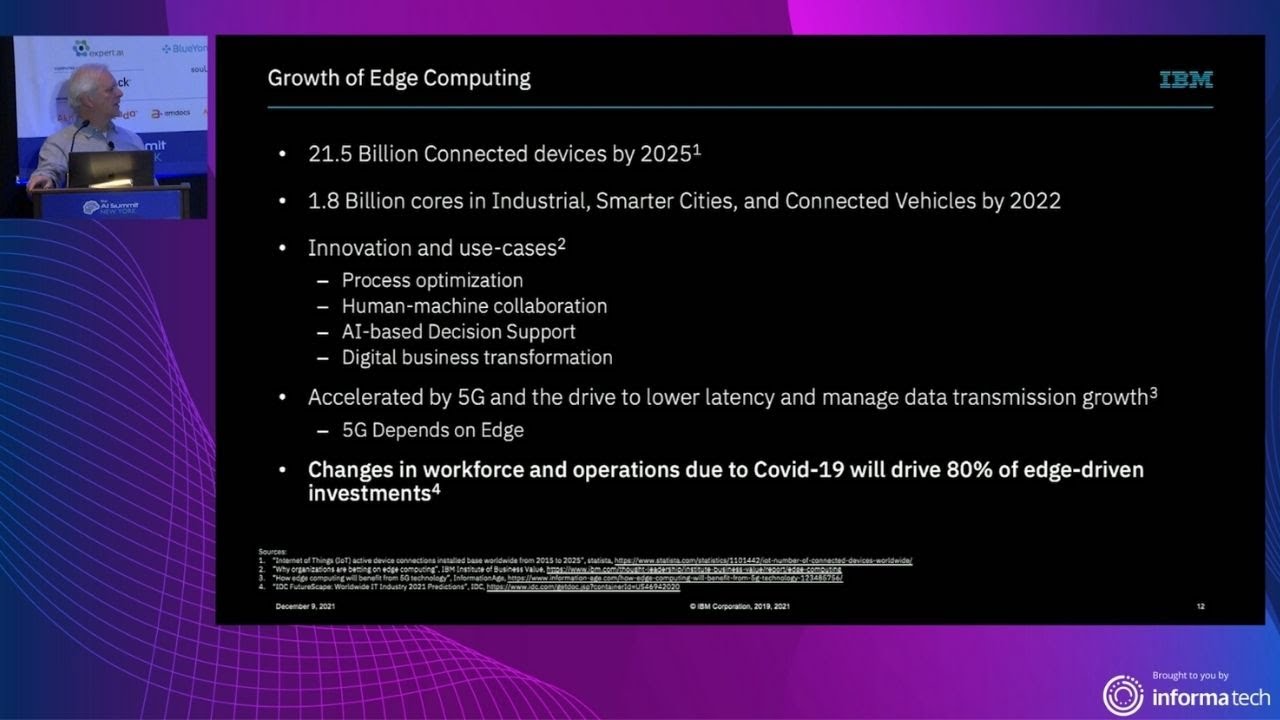
Why there’s a strong movement to get workloads closer to where people work.
It’s estimated that by 2025, 75% of enterprise data will be created and processed at the edge. In this video from the 2021 AI Summit in New York, IBM’s Rob High outlines edge computing market trends and what they all mean for your business.
You’ll learn
The three very distinct tiers and devices of edge computing
How edge computing complements and extends IoT
Why edge computing is critical for removing network constraints and restoring the benefits of cloud computing
Who is this for?
Host
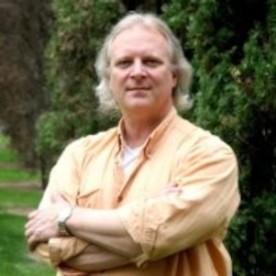
Transcript
0:04 all right so i'm going to talk a little bit about edge computing and i want to start with a few things that um i think are important and the first one
0:10 is that people want to make better and faster decisions
0:15 people also want to manage their operating costs transmission costs of course being part
0:22 of that but ingress costs etc are also part of their operating costs in any kind of
0:27 production environment people also are very sensitive to the personal private information and this is this is you know always i think for many
0:35 of us been true but it seems to be getting even more to the forefront especially with some of the things that
0:41 we've seen recently and ransomware and and other kinds of attacks like that but mostly just people
0:47 getting sensitive to the impact that that has on their business as well as on their personal lives
0:52 and then finally people are very concerned about how they maintain the continuity of their business and the presence of all this technology
0:58 and the potential for fragility in that technology and so for all those reasons we think that edge computing is becoming
1:04 increasingly important um but it's not just me i i think if we we'll see here in a few minutes the
1:10 analyst data about the marketplace really reaffirms that there is a strong
1:16 movement to get workloads closer to where people do their work
1:23 that's where the data's being created it's in uh it's on the factory line it's in the retail stores it's out in the
1:29 vehicles and the roadways that we travel that's where um we are as people that's where the
1:36 data that matters to us is being created either by ourselves or by the environment which we operate that's
1:41 where we make our decisions and the um
1:46 lowering the latency with which we can make those decisions the lower lowering the latency of the data processing that
1:52 allows us to make those decisions the ability to reduce the number of times that we might
1:59 be just transmitting sensitive information across the internet um
2:04 the ability to continue to operate even if somebody happens to dig up the uh the
2:10 network cables in front of our building you know any of those kinds of things uh begin to matter and edge computing goes
2:16 a long ways to helping that now when we talk about edge computing we like to talk about three very distinct tiers of edge computing the first of these being
2:22 um the network edge and eric called out the multi-axis edge computing
2:28 this the the mac as we like to call it actually services in a couple different
2:33 ways the first is within the network facilities themselves so whether that's a central office or a
2:39 regional data center or a hub location we're certainly seeing examples and occasions where
2:45 the uh the telcos the network operators are creating compute capacity in the network facilities
2:51 and of course you know that's not just about creating a new cloud experience it's really about bringing those workloads
2:58 closer physically to where businesses operate and therefore reducing the latencies associated with
3:04 that but beyond that there's also compute in those network in the um those remote on-premise locations
3:10 where people do their work as well um it is not unusual
3:16 uh or let me say it differently it's very common to find compute capacity in
3:21 a data room or sometimes even directly on the factory floor uh they may be industrial pcs they may
3:27 be half racks it may be appliances but there's a lot of compute capacity out there on the factory floor or in the
3:33 retail store if you ever go to your favorite grocery store and get a chance to go back to the manager's office there's a good chance you're going to
3:38 see you know five or six or seven pcs you know sitting there on the desktop in
3:44 the manager's office of your favorite grocery store they're getting a little bit more organized sometimes a little bit more
3:49 structured there might be a a a network room in the store that they put that stuff in
3:55 and a rack but nonetheless compute on the uh in the retail store is very common as we get to intelligent and
4:01 connected vehicles and the intelligent road side you're going to see compute actually in the roadside infrastructure
4:08 itself and so that's the second tier of compute it's in the remote on-premise locations
4:14 and we call this out distinctly because typically what we find there is i.t like equipment stuff that we would
4:20 all recognize as you know a server in any other form but the last one which i think is really
4:26 interesting are the edge devices and they're kind of alluded to this a bit almost all equipment that we deal with
4:33 whether that's in our work or our home there's a good chance that that equipment now is being built with
4:39 arm or x86 chips sometimes with small capacity and some of that's because of
4:44 if they're um if they're mobile devices and battery operated you know we do have to be sensitive to the power consumption
4:50 they have but also in those cases um it's just cheaper and faster to build
4:56 um your device if you've got a general purpose computer in there and you can run linux and perhaps a docker runtime
5:03 in the device itself and what i'm talking about here are things like intelligent cameras right there's a good chance of the cameras that we have here
5:09 in the room have a computer in it uh the industrial robots that you see you'll
5:14 see some examples of this later on when we talk about uh spot these are things that have computers in them
5:21 the stuff that we don't think of necessarily as being a computer certainly we wouldn't think of it as i.t equipment
5:26 we might think of it as ot equipment there's a good chance that it has compute in it and when we think about
5:32 the capacity that each of those represent and the location each of those have relative to where the data is that we're
5:39 generating where the work is that we're performing and the data that we generate each of those have a potential role
5:45 and we can now start thinking about how to get some normalization across that as i
5:52 said a lot of equipment that have computers built into them what we call edge devices
5:58 are already running linux they may be running docker um the edge servers that we talk about or
6:04 the mac distribute the i'm sorry the dedicated max the uh 5g private max that we see out there in the roman arm
6:11 locations are probably running some form of kubernetes certainly the um facilities that we see
6:18 the compute that we see within the network facilities are all running kubernetes generally speaking
6:24 and so what becomes interesting here is the idea that cloud-native development practices
6:30 can be applied for creating workloads that will run on any of these tiers including the cloud of course
6:36 right and when i say cloud here i mean of course public and private uh data center uh clouds whatever the forum is
6:43 but cloud native development practices seem to be um taking hold as the dominant form of creating application
6:49 workloads and we when you have that kind of consistency across all those tiers it really opens up to the idea of
6:55 multi-tier distributed computing applications which of course is not a new idea but it's an idea that's coming back
7:01 because of this normalization that we're seeing across all these different tiers and the ability to take the same
7:07 development practices that you would apply to building applications in the cloud can now also be applied to all
7:12 these other tiers as well and that becomes really important when we think about ai's here and you'll see that
7:17 surface more in a minute just to make a point how here however um
7:22 iot is a another term that you'll have very you'll hear very commonly when we talk about edge computing a lot of
7:28 people sort of think of edge computing as being born out of the it area and in some sense it is obviously what we're
7:34 talking about here are devices that can be connected and for which we can use internet protocols to access and communicate with
7:41 but there's an evolution whereas in the past iot devices were typically
7:47 you know built with asics they were relatively dumb or fixed function they had sensors and you would use them
7:52 to kind of connect and collect sensor data off of those iot devices that you might then transmit back to the cloud
7:58 today within the introduction of edge computing we can begin to process that
8:03 sensor data more locally if not on the sensor itself as i said some of that's now turning
8:09 into what we call edge devices merely by virtue of the fact that we've added a general
8:14 purpose compute capacity in there and have the flexibility of deploying other workloads we can now consider those
8:20 traditional iot devices edge devices but even when they continue to be sort
8:25 of you know if you will dumb uh sensor data devices we can still connect those to localized edge devices um or edge
8:32 servers etc um as i said the growth of edge computing is just phenomenal and this is
8:39 this is some gartner data that was published in a couple of different papers but uh you know if we presume
8:45 last year 10 of compute was done at the edge 90 is being done in cloud or i.t data centers
8:54 as we move into next year we expect that to be closer to about 50 and this is
8:59 this is not far off from what we're actually exhibiting uh in the field today already a tremendous amount of the
9:06 data that you create in these locations whether it's on the factory or retail or otherwise
9:12 is being actually processed locally in those locations as we move into 2025 we think that as
9:18 much as 75 of all enterprise data that is created in these locations will be processed
9:25 there and that's not to suggest that um cloud computing goes away if anything cloud computing continues to
9:32 grow and it's like growth is accelerated by the growth of edge computing this is a phenomenon that we experienced back in
9:38 the days of client server and again in the area era of soa and web applications
9:43 uh you know these kinds of technologies tend to drive a lot more transactional demand to the back end so we should
9:49 expect to see that here as well the difference being of course that when we're doing that we're doing that more efficiently what we're using the cloud
9:55 for is something that's more specific to the value that cloud processing brings to us but again the
10:02 point being that we've had this point of normalization and we examine the utility that each of these different tiers bring
10:08 to the table we can now begin to think about the trade-offs between cost and performance
10:15 we can think about where is the right place to put our workloads based on
10:20 the utility that that workload has and the dependency that workload has on things like latency
10:26 the further you get close to where the data's been created and where the decisions are being made
10:33 the lower the latency you can create by putting doing a compute there's a pretty obvious example but
10:38 but that's a that's a premise that we can exploit across every tier and now we
10:44 can right size the placement of workloads knowing that
10:49 these are not necessarily architectural assumptions that we have to bake into the solutions that we produce
10:55 we can in fact today decide to put workloads in the cloud that tomorrow we can change our mind and move that
11:02 workload closer either into the network or in edge server perhaps or even onto
11:07 the edge device itself or conversely we may over optimize
11:12 and realize and by placing workloads really on the device and then later realized that we have either competing
11:19 demands for that limited resource or the cost of running it there is um uh too high for the value that we're
11:26 getting from it and so then we can begin to migrate workloads back from the far edge back into
11:31 more near-edge locations and so for the first time i think in the
11:36 history of our information systems industry we've truly had the ability to make cost trade-off cost-benefit
11:42 trade-offs over the placement of workloads and be able to make that flexible over time so this is a key thing for us
11:48 to be considering and if you're wondering what i mean when i talk about edge computing devices i'm
11:54 just giving you a very small sample of the huge array of uh edge computing
11:59 capabilities that are out there on the market already today and you'll see here sort of a
12:05 sort of wide range of things that are general purpose compute and highly specialized compute in this
12:11 case hp retail systems these eurotech bolt gates are designed specifically for
12:16 industrial environments of course you can see things like industrial robots and intelligent power controllers so a
12:23 wide range of the kinds of things that have computed it today all of these things run linux all of them have either
12:29 docker or kubernetes runtime kubernetes platforms running in them today
12:35 and again i might emphasize this is just a very small sampling
12:40 where are we seeing the the application of edge computing is most often is around things like process
12:47 optimization situational awareness which might be for worker safety or things like that human machine
12:53 collaboration is becoming very important thing and in fact i'll talk a little bit more about this later on when we talk about cobots um of course lots and lots
13:00 of ai the most commonly referred to workloads that we hear today in the edge computing
13:06 space tend to be some sort of ai based workload whether that's visual recognition eric talked a little bit
13:12 about that whether that's acoustic recognition whether it's just stream data analysis
13:18 ai of various sorts are the kinds of things that we typically find out there and primarily because these things tend to
13:24 be very data intensive and the cost of transmitting the data is not only high in terms of operating cost but also you
13:32 lose the benefit of latency when you have to move all that data back up to the cloud process it there and then
13:37 eventually turn it back around if you have to suffer 100 400 500 milliseconds of latency that may be fine for human
13:44 interactions but when it comes to machine and in industrial processes and you're doing
13:50 you've got manufacturing processes that are putting out 100 widgets a minute you know half second latency
13:56 to make the decision whether to reject a quality problem is way too long right you need to make
14:03 these decisions much faster than that um we're seeing this uh being applied primarily in industrial manufacturing
14:09 scenarios secondly in infrastructure and why say infrastructure here i mean roadsides buildings you know maintenance
14:15 kinds of problems uh retail is a really hot area right now you see a lot of
14:21 interest in the retail industry especially as a result of covin i'll talk a little about that in a minute here too
14:26 to automate and reduce the amount of touch if you will in the retail set settings if you were a retailer you
14:32 certainly had to figure out how to get you know buy online pick up the store processes in place so a lot of retail um
14:40 of course you know the amazon go concept is taking hold in the retail industry everybody wants to kind of move towards
14:46 a more intelligent store automated check out kinds of scenarios in the retail space and of course
14:52 services um data is an ongoing i mean we've been
14:57 talking about in the ai world you know the growth of data for a long time here well this is very real and it's very
15:03 expensive people can't keep up with the rate at which new data is being generated and they can't process it they
15:09 simply don't have the capacity um the wherewithal to process it when you bring
15:15 tons and tons of data back into data center into your cloud it's great that you have this huge data lake but what
15:20 are you going to do with it and figuring how to build the analytics for that is is always a difficult task especially given the
15:27 specialties that we need around data sciences to be able to do that with the more that we can fragment the data
15:32 and deal with it in flight the more that we can filter it we can reduce it and get it down to the most essential and relevant data for us with within any of
15:39 our operations the earlier that we can do that in the process the better and so that's another reason why um uh inter edge computing is
15:47 becoming really important um i've talked a little bit about the growth just to put this in numbers 20
15:53 21.5 billion devices will be connected to uh the net internet by 2025 20 1.5
16:00 billion think about in the world of mobile computing right today we have something like three and a quarter billion mobile devices in the
16:06 marketplace today this is you know this is you know a multiplier number of devices
16:12 that will be connected and again you know these are not just dumb iot devices these are pieces of
16:18 equipment that have you know chips in them that you can compute on the reason why softbank paid what they
16:24 did for arm and why later nvidia has been so attracted to the idea of purchasing arm is because of the growth
16:31 of the number of chips that are going to be consumed in these kinds of devices over time
16:36 there are a lot of changes that are occurring in the especially the workforce and with round processes both in industrial but
16:44 also in retail and i hinted at this before largely due to the impact that kova 19 is having on the way that we do
16:50 business if you're a retailer as you as i said ever before you need to reduce your
16:55 touch footprint um with your clients the retailers that were able to stay in
17:01 business during the sort of economic crisis that pandemic uh introduced were the ones that gravitate towards
17:07 digitalization that you know introduce you know buy online and pick up the store pick up the curb if you go to
17:14 your favorite restaurant whether it's here in the city or anywhere else chances are you're going to be exposed to a qr code to get your menu
17:22 and of course that's just step one towards a more automated ordering system sooner or later we may not even have
17:27 servers uh in these in some of the best restaurants because everything will be digitalized
17:32 even in the restaurant if you're in the store trying to track you know spillage spoilage
17:38 um loss prevention any of those kinds of situations are requiring a more digital presence digital footprint in the store
17:46 pharmacies if you're a cvs or walgreens and you've got
17:51 pharmaceuticals in a refrigerator and somebody actually leaves that refrigerator door open
17:57 and the temperature inside the refrigerator rises overnight um you could easily use ten thousand fifty
18:02 thousand dollars of um pharmaceuticals just in that one incident so the cost of
18:07 batters is very uh the cost of uh these issues is really high um
18:12 if you lose connectivity to your store um you know some some retailers will tell
18:18 you it may cost them between 50 000 and 100 000 an hour while they are offline in that store
18:25 uh one of the obviously you know the the the not the ability you know if you lose
18:30 the ability to check people out you're losing revenue if you lose the ability to serve your uh clientele um you could
18:37 be impacting experiences uh creating uh issues for you for trying to get those reach those uh consumers
18:44 back if you are a grocery store and you um uh
18:49 you support um and i forget what the acronym is for it but basically food stamps or food vouchers
18:57 the law says that if you cannot check a person out and they're trying to pay with
19:02 food vouchers the food is free right so nowadays it's very expensive for retailers and trying to maintain
19:09 their productivity during that time is really key one example of the use cases that we see
19:14 today is the application of visual inspection and visual recognition for quality inspection um this in particular
19:20 this particular case we're using if red this is at a manufacturer that does welding if you're familiar with welding
19:27 processes one of the things that is always stymied the industry is how do you do
19:32 how do you ensure the quality of your weld activities typically in order to tell whether weld
19:39 was done well or not whether you have a quality problem or not you have to take um whatever it is that you just welded
19:44 and take it off the line cut it open and look inside or you might be able to do x-rays but even that requires uh taking
19:50 the quick the product offline to go do that very expensive process it's destructive
19:56 um obviously if you run into a problem because you're not going to sample every single car that you weld or every weld
20:01 that you perform you're going to take samples which means that if you had a quality problem that occurred earlier in your
20:08 cycle before you caught it now you gotta go remediate over all the different pieces of product that you involved
20:13 previously so in this case what we've done is we've taken an infrared camera we've trained it using machine learning
20:19 to recognize at the moment that it's being welded whether it's a good weld or not weld by virtue of the key signature
20:25 on that weld this as it's being performed now these are you know capturing full
20:31 resolution video to be able to do that in a typical manufacturing spot you may
20:36 have 100 200 400 robots each doing welding um activities
20:41 and if you have a camera assisted with each of each one of those you think about the amount of video data that generates you could easily over
20:48 overwhelm your networks trying to get all that data back up to the cloud on the other hand the amount
20:53 of time you're actually examining for quality is only a subset of the total time that those um that video camera is
20:59 on so you want to be able to turn it on and turn off the camera or at the very least you want to filter it because the only thing you
21:06 really care about is that one frame that indicated a failure a quality problem all the rest is interesting but somewhat
21:12 superfluous and if you do all that processing locally not only can you reduce the amount of data you have to transmit back
21:18 to the cloud you're still going to transmit the outcomes of that evaluation the inference results back up to the
21:24 cloud but that's a much smaller quantity of data than the actual raw video stream itself so we do that processing online
21:31 there in the factory we may actually activate into the ot system reject
21:36 processing and remediation if we do find a quality problem and then we report any kind of inferences that we got out of
21:42 that process back up to the cloud um the other thing to be thinking about here is
21:48 the data even even ais you know that we might consider here
21:53 whether that's for predictive maintenance whether that's for asset management monitoring getting the data has always been a
22:00 problem any of us who've been involved with machine learning know that getting the training data is always a hard task it's probably the
22:06 most significant issue that we have to deal with if you're in a factory that's been around for the last 20 30 60 100 years
22:14 you've got all kinds of equipment on that factory which is not digital it's hard to get the data off that equipment
22:20 you've got analog gauges you've got you know physical uh control valves and other things like
22:27 that you have to deal with it's not digital a very small percentage of the equipment on any given production
22:33 environment is digitized digitally enabled and so the question is how do you get data from a real world situation
22:40 when everything is analog so because of that and because of the the cost of um
22:47 upgrading everything to digital we've been working with boston dynamics because it basically introduces a way of
22:54 bringing data capture into the world in a very mobile and flexible way
23:00 we call it dynamic sensing and essentially taking a boston dynamics
23:06 spot robot in fact there's one out here i saw one at the deloitte booth um and putting sensors on it and then using
23:13 it to navigate across the production environment is a great way of overcoming so that some of those deficiencies in a
23:20 production environment where everything is um is analog we call these cobots because they're
23:26 collaborative they typically work with humans not not in place of humans uh
23:31 there may be some replacement in the case where humans today are exposed to arduous situations you know
23:39 it may be a safety benefit to run send a robot out onto certain production environments
23:46 um certainly there's a lot of heat there's a lot of noise there's a lot of other things that are unpleasant for humans to interact
23:52 with so there may be that but in any case these are typically working alongside
23:58 humans so we call them cobots this is an example of where we've applied it now we actually
24:04 took this video in the boston dynamics factory but we're working with a number of customers in
24:10 running the same use case in their environment as well in this particular case what we're doing is
24:15 using spot to go around and do fire extinguisher inspections
24:21 right so we know where all the fire extinguishers are across the factory this is the task that humans today have
24:26 to perform generally on a monthly basis they have to make sure that all fire extinguishers are in place that they are
24:32 not obstructed that that they're properly pressurized uh that the hoses and and so forth are uh
24:39 properly connected and so forth so instead of a human doing this task we can send spot out it will go to each
24:46 location now boston dynamics has done a phenomenal job giving spot the ability to navigate and
24:53 traverse in sort of unpredicted environments if you think about the the plant floor
24:59 you may know where you need to go but getting there is you know going to be exposed to all kinds of things that
25:05 you didn't anticipate like equipment being moved in the way or you probably saw earlier where spot stepped over over
25:12 another spot robot that was sitting down on the floor you know their ability to navigate around really you know tight
25:17 areas is phenomenal but what we've done here again is you know we've added a we've taken the camera on spot we've
25:24 added visual recognition um and to identify in this case that you
25:29 know the fire extinguisher is there but it's there's is obstructed there's stuff in front of it and what we do is we automatically issue
25:36 a work order um in this case through maximo to get a work crew out there to
25:41 fix that situation so this is a task that spock can do quite well
25:47 and um other things that we use spot for included oh wow time's gone fast okay other
25:53 things we use spot for is um to also look for we actually read gauges
26:00 so it can walk up to an analog gauge and read it and then basically transmit that digital information back um to
26:08 our work environment um okay i'm since i'm down to only two minutes um let me
26:13 hit a couple other points real fast one is we did this work with mayflower mayflower basically is a project to um
26:21 navigate from plymouth england to the united states in a ship that's fully autonomous there
26:27 is no human beings involved with this ship there's no crew quarters there's no space for anybody uh to be on board it
26:33 will navigate itself from one side of the ocean to the other uh again to be
26:38 able to do that we had to have compute on board it has to have the ability to recognize uh the obstacles it might encounter along
26:45 the way whether those are other ships or navigation things whether it's shorelines and rocks
26:51 and other things that you need to avoid uh sometimes there's debris in the water you know containers got you know fell
26:57 off another ship it has to navigate around and of course there's all kinds of marine life out there that you have to navigate around as well all that has
27:04 to happen on board um they there's very few cell towers at sea so uh
27:09 our communication is difficult through satellite and with very low latency low bandwidth um and so we can't kind of do
27:15 any kind of processing on site um and so i'm going to jump over that um
27:21 by the way one of the side effects of this is not just simply the technology of autonomous navigation but all the
27:27 marine science payloads that we put on board so if you think about the ship navigating the oceans
27:33 for weeks or months at a time collecting science data that can be then used for
27:38 you know evaluating the health of our oceans the impact of climate change etc that's an enormous benefit that we can
27:45 get in cost reduction if you want to learn more about the mayflower project you can go to mass400.com i'll put a qr
27:51 code there that you can capture that'll get you there too um all right let me wrap up here real
27:57 quickly with one last point we talk about edge computing but it's important to understand
28:02 that while we are leveraging cloud native development practices the edge is not the cloud there's a fundamental
28:08 difference between the cloud and the edge the cloud typically is centered around a lot of homogenous compute capacity what we're
28:15 looking for is lots and lots of services that all look the same so we can get elastic scalability over infinite resources virtually infinite resources
28:20 the edge is very different eric already talked about this lots of diversity of the edge a lot of dynamism the edge the
28:26 stuff moves around it gets repurposed it gets reconfigured a lot of security attack services at the edge because we
28:32 got people walking around the stuff it's not locked up in a uh a room with four walls and and uh armed guards and so
28:39 forth um and for that reason managing getting the right software to the right place at the right time getting the right ai out there and keeping up to
28:45 date keeping the models up to date is a task that we've taken on so my one plug here is for a product that we
28:52 built in ibm called ibm edge application manager its purpose is to get the right software the right place at the right
28:57 time that's what we do we do it at scale we can support up to forty thousand uh edge nodes it is based on open source we
29:04 uh we derive this from an open source project that we contribute to there's a vibrant community around it at linux
29:09 foundation called open horizon uh at the lf edge project one last thing i'm going to mention we
29:14 did some work with samsung we have modified the android kernel to
29:19 now host a docker runtime in android which means that all the things that we've been doing in terms of
29:26 containerizing ai and machine learning algorithms we can now also run on an android mobile device as well that's
29:33 still experimental right now we expect to have a formal release of that sometime next year but
29:39 this dramatically expands the utility of mobile devices in the world of edge computing and ai
29:46 so i'm going in there i want to thank you guys very much for your time sorry for going over a couple of minutes um qr
29:51 codes more information lots of links to other data that you can provide that you can look at to
29:58 learn more about what we're doing thank you
30:03 [Music]