Bob Friday Talks: Introducing Networking for AI
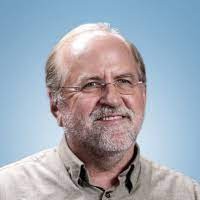

Networking for the AI Data Center
Join Juniper AI experts Bob Friday and Sharada Yeluri as they discuss how an efficient, high bandwidth, highly scalable network solution in the AI data center is necessary for today’s massive AI workloads.
You’ll learn
How frontend and backend data centers are used in AI
The advantages and disadvantages of using InfiniBand vs. ethernet
Why Juniper is committed to helping deliver interoperability standards
Who is this for?
Host
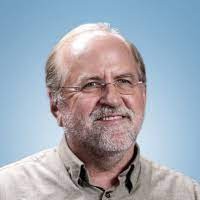
Experience More
Transcript
0:00 welcome to another episode of Bob Friday
0:02 talks we've been talking a lot about AI
0:05 for networking I thought we'd switch it
0:07 up today and talk a little bit about
0:08 networking for AI and for that I invited
0:11 Sharda from juniverse as6 Team to join
0:13 us today Sharda welcome maybe you give
0:15 the audience a little bit of background
0:17 on yourself yeah sure Bob uh so I'm
0:19 Sharda Yuri and I'm a senior director of
0:22 engineering in Silicon group I'm in
0:24 charge of building Express silicon
0:26 that's used in PTX family of uh routers
0:29 and switches that uh Juniper has been
0:31 delivering uh I have been in Juniper for
0:34 almost like two decades and I'm enjoying
0:36 every single day of it well welcome so
0:39 so maybe we'll start with you know AI
0:40 for networking networking for AI potato
0:43 potato maybe we start with you know what
0:45 is it different networking for AI how's
0:47 that relevant to what you're doing in A6
0:49 now yeah sure it's a little bit
0:51 confusing but it's not that hard to
0:53 understand so AI for networking uh it um
0:57 talks about like you know managing the
0:58 network using AI artificial intelligence
1:01 uh for example these network devices
1:03 they have become so complex these days
1:05 like you know root causing a problem
1:07 troubleshooting has become pretty
1:09 complex and what M and Mar have been
1:12 doing in the uh data centers Lan and Van
1:15 we want to move it to the data centers
1:16 as well and pretty much use AI to
1:20 efficiently manage the network like
1:22 troubleshoot analyze the traffic
1:23 patterns as well as like you know even
1:26 predict the future patterns whereas
1:28 networking for AI is the opposite of it
1:31 it is like uh using the network to run
1:34 the AI applications more efficiently so
1:37 while AI for networking is managing the
1:39 network using AI networking for AI is
1:42 actually like making the AI more
1:44 efficient using a efficient high
1:47 bandwidth highly scalable Network
1:49 solution okay so so this is what's
1:51 bringing Marvis to life you know some of
1:52 all this Marvis software is running
1:54 somewhere now maybe we'll go a little
1:56 bit because I know our audience you know
1:57 we we've all heard that Nvidia is the
2:00 third most valuable company in the world
2:02 now um selling thousands of gpus you
2:06 know I kind of roughly understand that
2:07 hey Marvis is running on a Google Azure
2:11 AWS data center you know what is the
2:14 difference now between you know these
2:16 data centers where Marvis has been
2:17 running for the last 10 15 years and
2:20 this new networking for AI data center
2:22 so the ones the data centers that we all
2:24 know uh we it's also we can call it like
2:27 a front-end data center because they're
2:28 fronting the users users can run
2:31 applications on the servers in the data
2:32 centers and lot of these data centers
2:35 are connected through high bandwidth
2:36 ethernet switches and Juniper has many
2:39 of them whereas the one uh that we are
2:42 talking about AI um uh workloads that's
2:45 a backend data center so that connects
2:47 many of the gpus like the ones from
2:49 Nvidia or from AMD you are connecting
2:52 them together to run the AI workloads
2:54 and here you can either use ethernet
2:56 switches or infinite band switches so
2:59 that's the difference so they're
3:00 completely isolated uh they don't like
3:02 you know intersect with each other so so
3:04 like when I started Miss I I built a
3:05 whole bunch of software built a whole
3:07 new Cloud architecture on top of AWS you
3:10 know did AWS have a backend back then
3:12 you know what's maybe a little bit more
3:13 detail of I got this front end I take it
3:16 that's been there forever now and is
3:18 this backend new or is there always been
3:20 a backend to these data centers yeah
3:22 there's always been a backend because
3:23 this AI artificial intelligence models
3:25 have been here for a while and the
3:28 models have been increasing in complex
3:30 both in terms of like the size of the
3:31 model and the training data sets and
3:34 they have been like you know you have to
3:35 split these models across the many gpus
3:38 the moment you have to split the model
3:39 across many gpus you need a uh
3:42 interconnect and then when you are
3:44 connecting them all together and that's
3:46 where the backend data center is but
3:49 recently with all this llm stuff like
3:51 you know all this generative a stuff the
3:54 scale of this uh data center has gone
3:56 like you know thousands of times uh from
3:59 what we were used to before so that's
4:00 where all the new challenges are coming
4:02 in okay so these data centers have
4:03 always had GPU somewhere in the back
4:05 closet we just didn't realize it and so
4:07 so maybe give the audience a little bit
4:09 you know Nvidia shipping you know
4:12 millions of these gpus what is causing
4:14 all those gpus usually now what what are
4:17 they doing with the gpus so the gpus are
4:19 mainly used for uh training in the
4:22 backend data center whereas the front
4:24 end gpus can be used for inference uh
4:26 the difference between the training and
4:27 inference is training you are taking an
4:29 AI model and you're running through like
4:32 an iterations of training so the model
4:34 can do what you want it to do whereas
4:36 the inference then you are using the
4:38 model and then you are like you know
4:39 giving a new input like you know for
4:41 example a prompt or anything like you
4:43 know when you are inferencing when
4:44 you're asking chat GPT something and
4:46 then you get the results out of it so
4:48 the inference ones are probably running
4:50 most probably running on the front end
4:52 side uh and that's where like you know
4:55 um uh it's like you know user interfaces
4:57 with them the backend ones mainly are
4:59 used for the training workloads um and
5:02 the way the training is done is because
5:05 these models are really large you split
5:08 them across many many gpus and then uh
5:11 you are running uh parallel computations
5:14 across all of them and uh once um all
5:18 these computations are done then the
5:20 gpus are exchanging the results and
5:23 after that they start the recomputation
5:25 again so that's where the AI workload is
5:28 little bit unique in terms of compar to
5:30 the front end workload because there's
5:32 lot of compute and lot of exchange of
5:35 like you know heavy um uh traffic that's
5:38 going on with low entropy between the
5:40 gpus okay so so if I got this right you
5:42 know if I think about the front end of
5:44 this data scenario this is like x86
5:47 Intel servers right this is if I think
5:50 of it Intel is the king of the front end
5:53 and invid is the king of the back end is
5:55 that the way you think about this I got
5:56 invid gpus you know so if I think about
6:00 your typical data center you know
6:02 ethernet has been the king of hooking up
6:04 all these servers and everything you
6:06 know what about this infin ban I hear
6:08 about you know I hear that when we get
6:09 into these high performance gpus you
6:11 know we have this little infiniband
6:13 versus ethernet going on so to answer
6:15 the first question yeah Nvidia gpus are
6:18 the most dominant ones that are used in
6:19 all the backend data centers although
6:21 AMD is also coming up with its own GPU
6:24 and there are some other companies that
6:25 are also coming up with the gpus and
6:28 these gpus can be connected
6:30 with either infin band or ethernet and
6:33 like so far like you know before this
6:35 ethernet everywhere thing that momentum
6:37 has started um they were mainly using
6:40 the infinite band switches and infinite
6:42 band is something that was um invented
6:45 to replace PCI uh for um high bandwidth
6:49 and low latency communication uh between
6:51 the storage devices servers and embeded
6:54 systems and melanox uh which was the
6:58 company that was um a standalone company
7:00 before Nvidia bought it uh it actually
7:03 like uh took the infinite band to the
7:06 next level by using it in its features
7:08 and Nvidia bought an melanox so it can
7:12 build an end to- end solution like a HPC
7:14 cluster or AI cluster with it and when
7:18 it comes to this data center grade
7:20 switches for infinite band that means
7:22 the switches that have very high radics
7:24 like you know very high throughput um
7:26 Nvidia is the only vendor so there is a
7:29 customer Monopoly there and then it's
7:31 pretty hard to like control the prices
7:33 so and that's why recently like you know
7:36 we are all trying to see how ethernet
7:38 can enter that market space and and we
7:41 are getting like you know pretty
7:43 successful in that market it sounds like
7:44 Juniper's team ethernet you know the
7:47 front end of this data center is all
7:48 ethernet you know Juniper space now on
7:51 the back end you know sounds like we
7:53 have Nvidia team uh Nvidia team
7:56 infiniband and we have Team Jupiter
7:58 ethernet
8:00 you know maybe a little bit about the
8:01 Gap you know when someone's trying to
8:03 make a decision between ethernet and F
8:05 band is there really a decision is the
8:07 gap really that big performance yeah
8:09 yeah so the reason for ethernet maybe
8:11 there are a couple of reasons it's not
8:13 yeah it's not only Juniper a lot of
8:14 other companies are also like you know
8:16 build building ethernet switches so
8:18 ethernet switches because it's
8:20 everywhere like you know everywhere like
8:22 you know from core like you know data
8:24 centers and L van all of it like you
8:27 know ethernet switches are there there
8:28 is uh a rich vendor ecosystem and that's
8:32 kind of getting the prices down and also
8:34 it's um also encouraging lot of
8:37 innovation so if you look at it the
8:39 highest performing ethernet switch on
8:41 the market today it has at least two
8:43 times the bandwidth than the infinite
8:45 band switch so that means you need like
8:47 you know almost half the number of
8:48 switches to build the same fabric uh
8:51 because your bandwidth is double then
8:52 you don't need that many switches and
8:55 coming to these camps like you Nvidia
8:57 versus ethernet I wouldn't say Nvidia is
8:59 just the infinite band camp they are
9:01 also building ethernet switches and
9:03 ironically their ethernet switches have
9:05 double the bandwidth than their infinite
9:07 vanand switch uh whatever is latest in
9:09 the market uh so coming to building an
9:12 ethernet switch um I I believe very
9:16 strongly that ethernet has come a long
9:18 way that I mean uh we have enough hooks
9:23 uh in place to build like you know these
9:24 large data centers with like you know
9:26 thousands of gpus using the ethernet
9:28 yeah well I'm a big fan of interoperable
9:30 standards and I think we've seen
9:32 ethernet adapt to a lot of different use
9:34 cases so I will tell you my money is on
9:35 ethernet going forward you know the
9:37 other thing I've seen you you've written
9:38 a lot of Articles and a lot of blogs
9:40 about you know putting these big large
9:42 llm on top of these GPU clusters you
9:45 know and about energy sustainability and
9:47 making sure we're using these gpus
9:49 efficiently you know maybe a little bit
9:51 of details I'm trying to understanding
9:54 know what's going on with all this
9:55 training uh I think I talked little bit
9:58 about the training before right so so in
9:59 terms of training uh like you know you
10:01 first like you know divide the uh model
10:04 into many many gpus like you know
10:06 depending upon the size of the model and
10:08 then you go through this iteration of
10:11 training so in at the end of every
10:13 iteration these gpus are all exchanging
10:15 results that's a very high bandwidth
10:17 communication then the next iteration
10:19 starts then they have to do the same
10:21 compute communication compute
10:23 communication um like Steps so the
10:25 communication part of it like plays a
10:28 very important role Ro so even though
10:30 people can claim like you know your
10:32 network switch is only like you know 15%
10:34 of the cost of the total data center
10:37 because gpus dominate the uh cost of the
10:39 data center uh the backend data center
10:42 if the network switch is not efficient
10:44 and it's causing congestion you're not
10:46 going to use the gpus efficiently so
10:49 even if you lose like 5% of um
10:52 efficiency in the GPU you need 5% more
10:54 gpus to do the same training right so
10:57 that's why the network uh um congestion
11:01 uh that can happen little bit more in
11:03 Ethernet compared to Infinity band like
11:05 you know how to control the con and how
11:08 to utilize the links uh between the gpus
11:11 so they are completely utilized are the
11:14 challenging things uh that come into the
11:16 internet uh uh switching like you know
11:18 when you're building the data center
11:19 switches with internet yeah you know
11:21 sort of you know standards
11:23 interoperability I am a big fan of
11:24 Standards inability they almost always
11:26 win you know maybe a little bit about
11:29 what is Juniper doing around standards
11:30 and operability for networking for AI in
11:33 these GPU clusters that we're building
11:35 sure yeah there's a good question Bob so
11:37 if you look at like the some of the
11:39 things I talked about before like you
11:40 know we need to improve the L link
11:42 utilization we need to control the
11:43 congestion so we are not increasing the
11:46 job completion time of these workloads
11:48 um there are different techniques uh
11:50 like you know for example if you want to
11:52 increase link utilization you can spray
11:54 the packets but unless the Nick can re
11:56 audit the packets the spraying is not
11:57 going to work similar Sly congestion
11:59 control there are different mechanisms
12:01 that each vendor is doing so the
12:03 solution space is little bit scattered
12:05 it's not truly interoperable maybe at
12:08 any point in time you can see probably
12:09 like two or three vendors that are doing
12:11 the same thing but not every vendor is
12:13 doing the same thing right so that's
12:15 where um this something called Ultra
12:17 ethernet Consortium uh that was uh uh
12:20 started and by hyperscalers and many
12:22 switch vendors and Juniper is a proud
12:25 member of the Consortium and the consor
12:29 is exactly trying to do this coming up
12:31 with a standard like you know enhancing
12:33 the ethernet so it can handle this high
12:35 bandwidth and uh low latency
12:38 communication and handle congestion
12:40 properly and all the other things that
12:43 you can do and uh the Moto or whatever
12:46 the main goal of the consultion is to
12:49 have a truly interoperable system so and
12:52 there are many working groups and we are
12:53 actively participating and uh one thing
12:56 we do want to see is that like you know
12:58 all the custom silicon that we are
12:59 building we want to make sure it
13:01 complies to the new standards that are
13:02 coming from the uh U Consortium well as
13:06 I said shter my money is on Juden per
13:08 and ethernet so thank you for joining us
13:11 and thank you for joining us until next
13:13 time have a great
13:21 weekend