Bob Friday Talks - AI Alchemy: Building Trust in the Digital Wizard
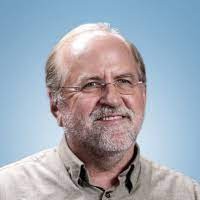

Building trust in AI
The main concerns around adopting AI are ethics, governance, and overall trust. This episode tackles common questions around these topics and covers the importance of explainable AI (XAI) to show that, compared to other industries, AI for networking is a relatively safe space.
You’ll learn
How to train AI models to possess the same level of ethics as humans
What XAI is and how to deliver it
Who is this for?
Host
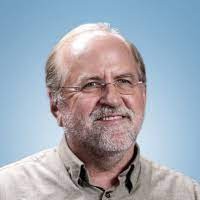

Experience More
Transcript
0:00 hi I'm emine and welcome to another
0:02 episode of poob Friday talks AI has been
0:05 associated with doomsday scenarios and
0:08 stories and pop culture however there
0:10 are more basic concerns about AI than
0:13 Terminators ethical concerns governance
0:16 trust become important so today I'm
0:19 joined by Bob Friday chief AI officer
0:23 here at Juniper to talk about the ethics
0:25 behind AI thanks for being here Bob y
0:28 thanks for having me so so this is a
0:30 very interesting topic so let's dive
0:33 into our first question why is AI
0:36 different than other intelligent
0:38 solutions that we have built in the past
0:40 yeah you know what I tell people is AI
0:42 is really the next step in the evolution
0:44 of automation you know and what's really
0:46 different about this generation of
0:49 automation is in the past we were
0:50 Building Solutions that were very
0:52 deterministic right you know the robot
0:54 that worked on your car did the same
0:56 thing day in and day out you know the
0:58 script that the network it guy wrote was
1:01 basically doing the same thing day and
1:03 out we're now building AI solutions that
1:05 are really doing Solutions on par with
1:09 humans right we're basically Building
1:10 Solutions that are starting to do
1:12 cognitive reasoning right and that is
1:14 why people are starting to look at the
1:16 issues around when you build a solution
1:19 that is basically changing Behavior
1:21 right just like a real person you know
1:24 when you hire someone that person learns
1:26 and changes his behavior and skills
1:28 we're building AI Solutions that look
1:30 and feel like an actual person or what a
1:32 human's doing and same ethical concerns
1:36 that we see with humans now are starting
1:38 to apply to AI when you actually see
1:39 their behavior change you don't know
1:41 what exactly is going to happen on the
1:42 next day so there are a bunch of uh
1:45 ethical questions around AI but to your
1:48 you know to your opinion in your opinion
1:50 what's uh some top concerns about Ethics
1:53 in AI yeah I you know I think what
1:55 people are looking at right now you look
1:57 like the self-driving car no we're
2:00 approaching a day some point where we're
2:02 going to find out that these AI
2:03 algorithms and self-driving cars are
2:05 actually safer than humans right and so
2:09 there may be a day when all of a sudden
2:10 you know driving becomes illegal you
2:13 know so there'll be eth and it's not so
2:15 much an ethical concern is it's going to
2:17 get more into liability you know when
2:19 you have a car wreck inherently you're
2:21 liable for that wreck what it's going to
2:23 happen in the days when you're south-
2:25 driving car wrecks who's liable is it
2:27 going to be you or the manufacturer
2:29 after the car so those are the issues
2:31 we're starting to face with these AI
2:33 solutions that are starting to do more
2:35 and more things that are really on par
2:37 with humans that we have humans doing
2:39 right
2:39 now Bob that's an excellent example on
2:42 the self-driving cars now as more Fields
2:45 let's say healthc care HR hiring OR tech
2:50 jobs more F to adopt AI how do we train
2:53 AI models to have the same level of
2:55 Ethics as humans and how do we ensure
2:58 that it's unbiased that it's fair and
3:01 who should be held accountable yeah you
3:03 know a great example of this would be
3:05 you know if you look at what we're doing
3:07 with chat gbt you know if I were to give
3:09 chat TPT the mission to optimize a
3:13 website for profit and actually give it
3:15 access to a bank account which we are
3:17 close to doing right now the same
3:19 business ethics that we apply to a
3:20 business would want to be applied to
3:22 chat TBT right if chat gbt is on the
3:26 mission to basically optimize this
3:28 website for profit and it decides that
3:30 it's going to put its competition out of
3:31 business you want to make sure that AI
3:34 has the same ethics that we apply to our
3:36 business standards apply to that AI
3:38 assistant right and if you look in our
3:40 HR departments right the same ethics
3:42 against discrimination right if we're
3:45 using an AI tool for hiring we want to
3:48 make sure the same ethics that apply to
3:49 that human HR department also apply to
3:52 that AI we don't want any discrimination
3:54 to be built into our AI Solutions in the
3:56 future same as we would apply to any
3:59 human situ ation MH now maybe let's deep
4:02 dive into AI governance can you expand
4:04 more on that part you know AI governance
4:07 is almost a topic similar to ESG right
4:10 you know where our customers are
4:11 starting to ask us what are we doing on
4:14 par with carbon right so when we get our
4:17 rfps a lot of those things we have to
4:19 explain how we're helping the global
4:21 world around lowering carbon emissions
4:24 we're starting to see AI governance show
4:25 up in that same ESG type of category
4:28 they want to understand what is our
4:29 policy and our governance and our
4:31 principles around Ai and adopting AI
4:34 inside of juniper Juniper currently has
4:37 a set of AI principles around the
4:38 network so the first step is really
4:40 making sure that you have a set of AI
4:42 principles when you're developing now
4:45 let's talk about trust we cannot really
4:47 adopt or work with a tool if we don't
4:50 trust it right so what is explainable AI
4:54 uh or explainability and how is it
4:56 related to trusting AI yeah another
4:59 great question and usually I probably
5:02 give the example of the solutions we're
5:04 really building or building doing things
5:06 on par with humans again and really just
5:09 like any other intern or new hire you
5:12 want to be able to understand what that
5:14 person can do you have to get to know
5:16 its skills and what it's capable of and
5:18 even more important like a human these
5:21 AI Solutions are going to start adapting
5:23 and changing their behavior as they
5:25 actually get more data and learn so that
5:28 is why I call the level of trust the
5:30 same trust we have to find in a new hire
5:33 is going to be similar to when I bring
5:35 on this AI assistance I'm going to want
5:38 to understand its skills what it's
5:40 capable of and I'm going to want to
5:42 learn that as it trust as it learns new
5:45 behaviors that actually it does not
5:47 change going into bad behaviors now
5:50 going back to training Technologies
5:51 right what measures do you take to
5:54 deliver Expendable Ai and transparency
5:57 yeah a good example of this is what
5:58 we're doing with zoom and teams right
6:01 now where we're taking Network features
6:03 we're taking Zoom data we're training
6:06 models to actually predict your Zoom
6:09 performance your latency right now what
6:12 we use now to explain what features are
6:14 relevant to those predictions is
6:16 something called shapl This is a data
6:18 science technique is once you've trained
6:20 a model to actually predict something I
6:23 want to understand what features are
6:25 relevant in making those predictions so
6:28 that is exact transparency
6:30 we're starting to help our customers
6:32 understand how the model is making
6:33 predictions and what network features
6:35 are really relevant in those predictions
6:37 thanks V for walking us through the
6:39 ethics of AI this is a very heated topic
6:42 and thank you for listening until next
6:50 time