AI Data Center Networks


Modernizing Your AI Data Center Networks
Modern AI data center workloads bring new requirements and design best practices for the network infrastructure. Join Juniper experts Michal Styszynski and Mahesh Subramaniam as they present the new GPU to GPU connect training cluster and inference fabric design options, including fabric-level load balancing aspects, ROCEv2/DCQCN, and DC fabric traffic engineering.
You’ll learn
About DC architectures for existing and modern workloads
The lifecycle of an AI DC network
More information about AI DC technologies
Who is this for?
Host

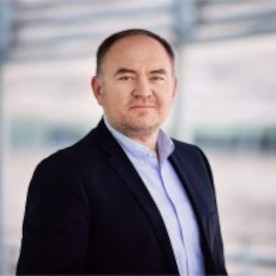
Experience More
Transcript
0:01 [Music] coming up next I would like to introduce
0:08 Michael stazinski and Mahesh subramanium who will be presenting AI data center
0:15 networks Michael is a senior project product manager at Juniper and is coming
0:21 to us today from France it's his second time presenting an anog Mahesh is
0:26 director of product management at Juniper he's coming to from the Bay Area and it I believe it's his first time
0:32 presenting at anog it's a pleasure to have them speaking with us
0:40 [Applause]
0:50 today by the way Mark Johnson it's a very interesting presentation and thanks
0:55 a lot for inspiration and lot of uh insight information starting from
1:01 internet node to quantum physics thank you very much for that by the way I mahes subam uh my colleague Mikel from
1:10 uh Juniper Network sta Center product team and our main um chatter there is to
1:18 focus on uh platform software uh specifically into automated secure data
1:25 centers and also the main idea of our thing is to a data CER Fabric and in
1:31 fact that is our main focus of the sessions too so uh to start with I would
1:36 like to make a kind of a statement here that if you talk about any 100 Gig GPU
1:43 clusters or even thousand even 10,000 32,000 the technology what we are going
1:51 to uh procure or we are going to adopt is ethernet why because ethernet is kind of
2:00 appr proven everybody knows here and also that there are two main reasons uh
2:05 with the latest data point I checked it in the internet also there are 600 million
2:11 ethernet switch ports has been deployed in L year uh with multiple vendors which
2:18 means we have a strong multivendor capability in the ethernet the second is
2:24 that strong intable capabilities because of the multivendor there is no vend lock
2:30 in so the strong interal capabilities which ethernet provides uh that's what we are going to
2:36 talk about now and with that said like uh we want to talk about our different
2:42 idea of architecture about a data center fabric also we would like to share uh in
2:48 the globally how we are solving the customer requirements in the a data center perspective that's what it's
2:54 going to be okay thank you so aenta going to be like
3:01 uh we are going to talk about uh DC data center Network existing workload how
3:07 it's going to differ from a data center Network Fabric and uh and also that uh
3:14 you would like to uh what is so why so special why it's now that's a main topic
3:20 going we are going to discuss and the second we are going to talk about the life cycle of a model right and how DC
3:27 data data center Network going to useful in the life cycle of a training models right that's second one and the third
3:34 one we are going to talk about the different DC Technologies how we are going to connect the gpus how we are
3:40 going to build the data center cluster uh connect together with the various type of connectivity that's going to be
3:46 third topic and fourth topic going to be key takeaway what we learned and what we are working behind the story about a
3:53 data center fabric okay uh I don't know uh in my
3:58 different presentation and conference in internal Juniper the statement what I'm going to make now it's going to be
4:04 cliche bear with me but it's very important when you are going to design
4:09 the data center in my perspective first we need to understand the behavior of the workload when I'm talking about the
4:16 behavior of the workload when we Define the workload Behavior it will Define the server requirement when we Define the
4:23 server requirement it will Define the server connectivity when we Define the server connectivity it it will Define
4:30 the data center design requirement of course that any data center workloads whatever you're seeing in the 10
4:36 workloads here behind everything going to use the claw architecture like leaf and spine no changes and it will be the
4:43 same claw architecture we are going to use it in even the 5G data center or in enterface data center of course in the
4:49 AI data center then what will be the different that's the different we need to understand that's an if you want to
4:56 understand the difference that means we need to understand the workload types for example if you're using a Enterprise
5:02 data center mostly you will use the evpn VXL because of multi- tendency and also
5:07 you need to have a data center interconnectivity you can use the vx to VX teaching there are lot of few
5:13 requirements are required on the same side if you're talking about 5G data centers there are 5G core component also
5:19 5G orand component like ODU ocu if you're talking about ocu if it is in the
5:26 4 data center that means the requirement are slightly different if the for data
5:31 center with the ocu you need a PTP syy timing is very important in the data center switches and other hand if you
5:37 were talking about remote da distributed a architecture for cable side you need a one x requirement in the switch so there
5:44 are different different requirement so we need to understand the behavior of the workload when you're talking about the
5:50 behavior of the workload out of all the 10 different workloads there are many but 10 to 12
5:56 years most of the data center workload evolvement there are many I would say like we pointed out around 10 here out
6:03 of this 10 nine were cloths other than
6:09 a using same claw architecture of course but it is not there are lot of
6:17 arithmetic calculation but less CPU intensed it's not highly CPU intensed that's a one difference second this
6:25 arithmetic this all the nine workloads are not fully interdependent with other
6:30 component of the system this is the key difference between other workloads right but when we talking about AI workload
6:38 right the a workload that the it's fully dependent with the system that means the
6:43 GPU has to communicate with other side of the GPU or inside the GPU sequenc has
6:49 to be connected that is tightly coupled not Loosely coupled the second there are petabyte of data intense arithmetic
6:57 calculation going into the GP servers to train the model for that CPU will not be
7:03 enough you need some kind of a parallel processor and also you need a GPU or TPU
7:08 that tensor tensor flow Processing Unit or the general Processing Unit or graphical processing anything you can
7:13 say but you need a GPU or TPU than CPU that is one thing if and what is the
7:21 other key difference out of all this nine workloads other than a workload the
7:27 payload is like a common evpn VX or IP fabric but in AI workload that is so
7:34 that's easy actually the only one workload we need to look into that only one workload traffic type we need to
7:39 look which is rdme which is uh remote direct memory access right this is the
7:45 key difference and with the RDM workload type how it behaves and how we are going
7:52 to connect the GPU cluster to move the memory Chunk from one place to other place that all we are going to see in
7:58 the next few sessions so this is so special because why it's now that main question the GPU is there
8:05 for long time I would say like like 10 years it's there right why it's now and
8:12 that's a main question we need to solve uh to do
8:17 that uh I would say that uh earlier there was a narrow Ai and N means you
8:25 can see your home alert system it will identify the person input output that will be sequential combinations if it is
8:31 any human it will give a ring alarm or it will give some alert it's a dog or cat something very simple narrow a
8:38 algorithms and even the spam filter you can see it in the email inbox you will see it but nowadays we are talking about
8:46 gen AI this is this is also same algorithm but it's a large language model or large video classification or
8:53 our audio classification we are doing it in that in gen AA it depends upon whatever ever the input you are giving
9:00 it will calculate that it understand the input and it will give the output accordingly that's another gen Evol and
9:08 other one then nowadays we talking about domain specific a that's domain a and other one is the AJ these are lot of
9:15 different different a models are coming in it BEC so interest for this feature and another one I want to
9:22 mention it that user interest after the chat gpt3 like one one or two years back
9:29 the interest becomes so intense I would say like a lot of people wants to know what it is and the applications are real
9:36 when we are doing some calculation and competitive analysis and even some business requirement there are 46 to 53%
9:44 of kager improvement for this a network which means the common annual growth rate the investment the companies are
9:50 putting together for a clust is real uh that's the reason we started working on two years back and we building a lot of
9:57 fabric technology for the same right
10:02 and also the server uh technology improved a lot we
10:09 are talking about the normal blade server and also nowadays there is a dgx HX h100 a100 different different servers
10:17 right not only that and in the server inside there is a PC bus right right now
10:23 the PC 5 it can go up to 30 GT per second that is 30 gig transfer per
10:29 second and even PC 6 we are looking at around 64 G GT per second this is huge
10:36 Improvement on how we are going to communicate inside the system on the other hand that is nmv that nonv Memory
10:44 Express that how you are moving the memory Chunk from one place to other place in the flash memory even you have
10:50 all your have laptop and it's booting so fast why because solid state memory and
10:55 so this the memory accesses become so fast and the crossing between the
11:01 internal perel become so far because of PC so server evaluation also went so
11:07 high so it's a right time and this is the correct time to go into that
11:12 training model and user interface is so high so we started building lot of traction lot of customer requirement lot
11:19 of customer R fees to how to build this cluster in my company it can be starting
11:25 from low end and it can go to high end also like what what I mean is that it can be start with 50 GP cluster or it
11:32 can go up to th000 GP cluster depends upon the customer requirement you want to add anything in
11:38 this no as a matter of fact I think I the especially the fact that users like
11:44 the application side of things is uh is crucial here right we can have beautiful Technologies but as long as there's no
11:51 adoption on the user side then it's not going to work uh so just as an anecdote
11:56 last week I was in in KH in France at the AI conference World Conference and
12:01 uh believe me guys this is huge right the number of startups building applications around this topic is huge
12:07 so it drives also the networking side of things as well right yeah so that's that's why we wanted to bring this topic
12:13 uh during the Nana conference yeah right yeah this uh the the last few slides
12:19 it's a literally staging slide uh we would like to uh make sure that why it's
12:25 so special and it's real and why it's now and but this is the main slide I
12:31 would say like how we are why RDMA traffic right and why we need a high Ric switches why
12:38 you need lossless fabric that are a very important question and to Define to
12:44 justify those questions we need to understand how this uh large language
12:49 models or that the how the training models works it's very very important
12:55 and as I said the Char gpt3 is the one of the main factor there are lot of Paradigm Shift about our understanding
13:02 our interest in the in the in the whole industry about AI because of that chat
13:08 gpt3 so we'll take that chat GP is one of the example so you have a lot of data
13:14 raw data maybe you can say it Wikipedia or you can say it take it in stock
13:19 exchange lot of datas are coming together right the raw data how it going into the training model so what they
13:26 will do they will take the data for for example chat GP they taken about the raw data and they tokenize the data they
13:33 label the data and there are lot of encoding mechanisms are there for example bite pair encoding one of the
13:39 typical example they will take the raw data they will make it as a data set the
13:45 data set will become a token token is nothing but like kind of a sequence integers like for example data center
13:52 means data will be one token Center will be another token data center will be the
13:58 third token token like the combination that's a unique integer and that will go into the training model that's the
14:05 reason you need a heavy lifting mathematical calculation or flops the Flop uh the floating Point operations so
14:12 it is so intense so we need a GPU for that and this they will take the raw data again go to chat gpt3 they taken
14:20 around I searched in Internet it's around 175 billion parameter going into
14:26 the training model and it took 30 days to train the model and you know they
14:32 have used Nvidia V100 server around 10,000 GPU servers they used to train
14:37 the model for 30 days likewise if you talk about llama that large language
14:43 meta I think they took around 21 days I'm not sure but then I think they took around 21 days so you need to take the
14:49 data you need to pre-process the data and which means you need to make toonize the data and we need to give it to the
14:56 GPU cluster that's point one and per GPU for example if you're talking about
15:01 Nvidia h100 that's a Talk of the Town it's per GPU will have 80 gb but I'm
15:07 talking about petabyte of data and how you are going to feed all the data into the one server it's not possible that
15:13 means you need thousands of servers thousands of gpus to feed the paby of uh
15:19 data the parameters or tokens into the GPU to train the particular parameters
15:25 to get the the preferable outcome or whatever the outcome you need it that's
15:30 what and if you have a thousands of gpus I mean 100 thousands of gpus or 100
15:36 thousands of gpus that means you have at least hundreds of servers per server right now as as I'm standing now it's
15:43 around 8 gpus per server that's what Nvidia providing it how you are going to connect those servers together you
15:49 cannot put all the Thousand or 100 service in one rack right so you need
15:55 multiple racks when you have multiple racks multiple servers automatically naturally the data center
16:01 fabric is vital so how you are going to connect the one GP to other GP from another rack that's where the fabric
16:07 coming into the picture and once it's trained the model the outcome we'll call
16:13 it as a gradient and the gradient will be be propagated to another GPU as I
16:19 said this a uh uh training or a cluster is sequency connected if one piece of
16:26 missing data outcome of the particular training you have to run the whole training again right we'll call it as a
16:34 Epoch or EPO or iteration there are lot of different different jargons are there I'm giving in the pl language if in the
16:41 iteration in one particular job one of the thread or one of the token didn't do the calculation properly didn't transfer
16:49 the RDM mam Chun from one place to another place you couldn't transfer that means you need to run the job whole job
16:55 again that means the CH GPT what I said 30 days it will become 60 days or more
17:00 than one year so the fabric lossless fabric is very important how you're moving a data from one place to other
17:07 place without any congestion without any packet drop is crucial that's the reason
17:13 data center coming to the picture it's not only stopping there the output of that particular uh the training will go
17:20 into the inference that's where the money is so wherever you are going to chat gpt3 you're typing in open AA
17:27 that's inference that's what you're seeing in the front end inference is not a very magic word it's very easy
17:32 whatever the data center fabric you have wherever you are hosting the web servers and Etc it's same thing right you are
17:39 getting the output you are placing in front of the multiple users and they will log in they will subscribe will get
17:45 the money for it so training is kind of a Apex and Opex and the inference is
17:51 that's where you are getting monitoring all those things we are getting business go to the second one yeah thank you so
17:58 the this is again uh this is a very high level slide Gathering the data train the data and
18:05 put it in front of the users uh whom they use it right for
18:11 example S3 open window you can open a.com you can get that one that's inference so uh go to the next
18:18 slide so what I'm trying to mean here is that Gathering the data that means you
18:24 need a storage cluster training the data that means you need a training cluster
18:30 and projecting the output you need a inference that means you need a inference cluster so whenever we are
18:37 talking about AI data center fabric it's not only one type of training data
18:43 center there are at least three to four different kind of a data center we are talking about one is the storage data
18:49 center second is the training Data Center and third one is the inference data center sometimes in the inference
18:56 they will use a share storage data dat Center as well and so there are four different kind of a data center we are
19:02 talking about here and uh go to the next slide this is kind of a a high level
19:09 view of the data center topology and if you're talking about storage inference
19:16 and training data center cluster how you are going to connect the servers in the training cluster is very important
19:23 because we already know how to solve the problem how to complete the use cases in the storage Data Center and we know how
19:30 the inference cluster also will work because already we have a lot of data center we are hosting lot of web servers
19:36 in that that's not an issue but in the training cluster is new how we are going to handle the RMA traffic how you are
19:42 moving from memory Chun Plum Chunk from one place to other place with a loss less with a less uh low latency I mean
19:50 say low tail latency to complete the job so that is very important so so we are
19:55 focusing only about training data center cluster the training data center cluster with available server connectivity now
20:01 go to the next slide there are two types we can connect the servers into the data
20:07 center one we are connecting all the AG gpus in the server to all the leaves
20:14 that's point one or all the H GPS in the server connecting to the one leaf not
20:22 eight Leaf so we will call it as a s o the stripe optimized design or we will
20:28 call it as a stripe unified design the stripe optimized Design This is what in Nvidia calling as
20:35 a rail optimized design there's a lot of good Advantage because the collectives
20:40 we call it that collectives means like when the training model is completed the GPU memory chunk will
20:48 communicate each other within the server we'll call it as nccl nickel on AMD will
20:53 call a rickle how we communicate each other within the server once it's completed that memory chunk information
20:59 will go to the other server that's called Collective Communications how we are doing it with the optimized design
21:07 if you notice properly in this diagram all the gpus from different different
21:13 server will connect to the different leaves which means each GPU will connect with each Leaf out of eight Leaf if any
21:20 failure happened the churn of the network will be high that's one of the disadvantage in the rail optimized I
21:27 would say the stripe of optimized design the second right now it is 8 GPU what
21:32 about 16 GPU per server or 32 GP per server that means your rack length will
21:38 be high your Optics connectiv Optics connectivity will be different how it
21:43 will different because that SI the length of the for example sr4 you using the optic connectivity for the short
21:49 range for rxes is so high that means you mainly you to use VR VR sr4 or LR4 lot
21:56 of different different Optics you need to use it in the switch and also you have want to add anything about multi tency in fact so in some situations
22:03 multi-tenancy may may be U well optional uh sometimes it's needed in order to monetize on the infrastructure so uh if
22:11 we want to extend the multi tency from the server itself to the network uh
22:18 there are different options one of the option is to leverage still the evpn VXL
22:23 using evpn route type 5 or the second option is to use some of the uh traffic
22:29 engineering capabilities in order for example to isolate uh the large language
22:34 models strip to stripe communication from the rest of the of the tenants so for example we may have a situation
22:41 where uh red tenant is very specific is using a lot of bandwidth and the flows
22:47 he's generating are very long live flows right so in this context we may be
22:53 tempted to use some of the traffic engineering mechanisms yeah that's true and and uh the another one is The Strife
23:00 UniFi design right the blast rate is so high there because the every GPU on the
23:08 particular server again eight gpus will connect to the only one leaf which means if Leaf's gone the server gone and then
23:15 blasted is so high but uh in this one the network churn if the failure
23:21 happens the churn will be less that's one of the advantage the Optics different type of Optics not required we
23:29 know what OBS we are going to use in the server specifically this kind of a design will be useful for like kind of a
23:36 uh if you have a high radic switches with the model chassis this kind of a uh
23:42 topology will be helpful what I mean is the high rics model chassis few customers um specifically in I think in
23:49 Japan they want to use a model chassis U and uh for example 576 400 gig port in
23:58 one chassis and all the gpus will connected to the one particular product
24:04 and that product that means the gp2 GP communication will be so fast and of course it will be lossless and that that
24:12 kind of Advantage we have it which means if you are going with some kind of a domain specific 50 GPU cluster or 100
24:19 GPU clusters this kind of a topology will be useful one off the point and so to summarize
24:26 this of course you know why we need a data center fabric for AA but the fabric
24:33 the GPU how we are going to connect there are two parts one is that stripe unified design stripe optimized design
24:39 there are pros and cons and depends upon what is your server and GPU cluster
24:45 connectivity and uh I want to summarize everything together because we talked
24:51 about a lot of things and lot of jorgans at the end the a data center requirements are
24:59 like we can talk it five parts one is that you need a high radic switches if
25:06 you have existing data center switches with a 25 gig or 100 Gig will not work I
25:12 can confidently say that because the GPU servers not like a CPU
25:19 servers the GPU servers the Nick connectivity itself like a 400 gig for example nvdia djx system having a CX7
25:27 the CX7 is the Nick itself with 400 gig how you are going to connect the Nick into the leaf that of course by default
25:33 you need a 400 gig so you need a high radic switches like 64 into 400 gig 64
25:40 into 800 gig and some people even we are talking about 64 into one Tera so those
25:45 kind of a high Ric switches you required that is the first requirement the second requirement is the lossless fabric
25:52 that's what the whole session going to be next M you are going to talk about it that the lossless fabric
25:59 means I told that it's very sensitive RDMA traffic it should not be any drop even single drop you need to run the job
26:06 again whole uh training model again the whole way so you need a lossless fabric
26:11 how to achieve the lossless fabric there are two ways you can achieve the lossless fabric one is the efficient
26:17 load balancing second is that congestion control right and efficient load
26:22 balancing there are different types like SLB DLB and now we are talking about glb also and the congestion control of
26:28 course the famous one is the rocky V2 where you will talk about the PFC ecn
26:34 how we can tweak it even we started working with the different ietf standards with the different vendors and
26:41 uh we are coming at a lot of different different ideas one of the idea is a source flow control where uh instead of
26:47 using the PFC we can use the source flow control one to uh I think qdw is one of
26:53 the standard how you can understand the congestion and how we will in inform the congestion information to the source
26:59 slow down the traffic flow and we need there is a congestion is there right there are different different method we
27:04 are going to see I think next uh half an hour you are going to talk about those things right exactly thank you so thank
27:10 you mahes so uh we've seen so far the architectural side we've seen the type of applications that we we we can run on
27:17 the on the backend Network on the front end uh so far you probably experience mainly the front end part and for the
27:24 rest of the session we'll focus on the on the backend side of the of the architecture so uh speaking about
27:31 the RDMA workloads uh mahes mentioned that the speed of processing is key here
27:37 so you can see on the on the diagram uh we don't have any kernel and the CPU uh
27:43 engaged in order to place the uh uh the data chunks on The Wire right so we can
27:51 directly process the chunks of data put it on the on the KN card and then send it to another server on the GPU side
27:58 right so here is the example just of the communication between the two of the servers crossing the uh the spine
28:05 devices the topology itself is very simple but obviously the number of uh the leave devices inside the topology
28:12 will depend on the on the number of the gpus that you have in your server or sometimes on something else right uh
28:19 what what is quite consistent is the speed of the connectivity so we you see on the diagram that it's a 400 gig
28:25 connect maybe 200 gig connect so this is the perfect use case of where we see the 400 gig 800 gig adoptions are really
28:33 really evolving very fast comparing to what we've seen so far in traditional networks right the adoption of 400 gig
28:39 is huge there and of course the number of ports of 400 gig connect is is growing very very fast so what what you
28:48 can see on the on the slide is that these memory chunks you can see on these uh Red Blocks are moving across the
28:55 network but they are moving thanks to the trans transport right specific transport we can see on the diagram that
29:01 the transport is an Ethernet on the outer side but then we can see that it's an IP UDP right UDP with the specific
29:08 destination UDP port and then some random Source UDP Port right but if I
29:14 have this uh fixed destination UDP port and some randomized uh uh Source UDP
29:20 Port uh for some uh uh situations we will see that it's not enough to efficiently load balance the traffic and
29:26 I cover that later during the session as well and then you can see the uh infin band the bth header that's the new stuff
29:34 which uh uh in case of networking we can use in order to actually process uh some
29:40 of the information on the switch itself and then uh take uh this information to
29:45 load balance the traffic based on the variations of the informations inside the the op code right like for example
29:52 we can decide on which path to send the traffic based on the type of operations we we are having either the read right
29:59 we can decide based on that uh how to uh how to in fact process the data on The
30:04 Wire right I want to add one point here um this is very important one uh about
30:10 that uh RDMA traffic right whenever you just Google it even now they will talk
30:15 about elephant flows right or jumbo frames and uh that is one thing to handle in the data center the second is
30:22 the less entropy which means elephant flows with a less entropy that is the biggest behavor change compared to any
30:29 other workload what you're seeing in the nine workloads this in& ml workload is 100% is RDM memory Chun transfer and in
30:37 that the RDMA traffic is like a like a like a elephant flows with a less entropy how to identify the entropy here
30:45 is that biggest challenge uh that's what we are solving it here so it means I can have a single Source UDP and then that
30:52 session can stay like for a long time right it just sends the data data on and on and on for for weeks sometimes and it
30:59 just it's a lot of bandwidth right so we need to dig into the packet and then uh use something else than just the uh the
31:05 transport UDP uh layer to actually forward the packets efficiently across the network right so we can either take
31:12 the the the the sequence numbers or uh uh the up code values to to take some
31:18 further decisions right so I spec I I was talking about the read write
31:23 operations so that's part of the uh communication between the servers uh and
31:29 uh what what is important that before we put the the data on The Wire uh there is
31:34 a a negotiation of the session that will take place so you see that on the on the
31:40 top of the uh session establishment uh summary diagram where we actually
31:46 exchange the information on the CER values right the CER values from the client as well as from the server are
31:52 exchange and then they are used at the transport level of the packet we've seen before right so that information is uh
31:59 is is crucial at the very beginning of the of the session establishment then once the client and the server they
32:05 agree on the these values they can decide to okay but what about the memory information which regions on the on the
32:12 memory I will be using in order to uh write the data too right so that that's
32:18 the second part of the of the session establishment and only then the data uh
32:23 transmission will happen right so what you can realize on this diagram that
32:28 there are acknowledgements right so the reability of the of the communication is maintained not at the UDP transport
32:35 level but at the a dma level so these acts are coming at the upper level right
32:41 from the RDMA uh stock itself right so we have a guarantee that the memory
32:46 chunks that I was showing will arrive uh for the given session in a reliable way
32:53 and so once the the job is terminated there is obviously a a a a graceful uh
32:58 uh termination of the session and we can see that at the bottom of the of the of the communication slide right so the
33:04 state machine is pretty well defined uh the communication is reliable uh these
33:09 are the the main points to to remember right and this this CER values are randomly actually uh initiated it's like
33:17 a think about this like a cue of of the jobs that needs to happen between the server and the client right so that's
33:24 that's what we wanted to highlight and then okay great we have a transport part
33:29 which uh is actually uh the part where the data is being exchanged but what if
33:34 in our network uh there are some problems in terms of entropy that mahes mentioned right then in some situations
33:41 we need some specific uh uh congestion management uh inside the three-stage
33:48 topology or five-stage on the diagram you have a five-stage IP claw topology and we see that uh on the supine two
33:55 there is a congestion point this CP is a congestion point where the rocky V2 data
34:01 actually flows to the server in the other pod in the Pod to but unfortunately there are other type of
34:07 communications which are congesting that link on the et0 interface so in that
34:12 situation we need to react on this right so uh there are two options of uh
34:18 managing such a situation one is to Simply uh tag the pockets on the data
34:23 side uh saying that the ecn explicit congestion notification uh changes the
34:29 state to beats values one one and so the server four in the Pod two will actually
34:35 realize okay well there is a there is a problem we have so I will inform the guy
34:40 who is at the region of the traffic and we'll ask him to slow down a little bit for very short time in order to avoid
34:47 the congestion on the on the CP congestion point so that's one option right and then once the reaction
34:54 happened on the server one we realize that okay but should we also use in
34:59 parallel the other congestion man management mechanism which is the priority flow control right so the
35:05 priority flow control is not something completely new it existed even in the L2 ethernet trunk type of topologies the
35:13 new thing that we are using here is is really that the uh priority flow control is set at the IP level and so this is
35:22 the second actually stage of notifying the originator of the data about the
35:28 congestion the reality is that sometimes you need to coordinate these two mechanisms so uh usually from the
35:34 implementation perspective it's the ecn that is uh triggered first on the switch
35:41 uh and only then eventually the PFC we may have also situations where only the ecn is used right the PFC Cascada effect
35:49 you can see on the diagram where uh uh the super spine sends the PFC packets
35:54 down to the to the origin in inside the the Pod one is not something necessarily people like because simply it just slows
36:02 down the other Communications right yeah and in this context the ecn is only used
36:07 uh uh in uh in the in the context where the the the PFC uh pause frames will be
36:13 triggered often there are some mitigation mechanisms that we can highlight as well where for example uh
36:19 there is a there is a function called the PFC watch doog where we can simply ignore these these push backs sent in
36:26 the pod one with the pfcs and then uh resume the traffic uh uh immediately
36:32 right but these two mechanisms the PFC as well as ecn they are the contributing factors for the situations where in fact
36:39 that load balancing I'll be talking about is not so efficient right so uh would you like to add anything on this
36:46 no that yeah handling this congestion is very important uh how we are one is that
36:51 in a traffic avoid the congestion or control the congestion right here we are
36:56 talking about controlling the congestion when you are trying talking about avoiding the congestion that means you
37:02 are talking about some kind of a schedule Fabric and etc etc with other vendors are using it right and uh few
37:08 vendors are using it I would say in scheduling fabric we are having a virtual output queue you will hold that
37:14 RDMA traffic you look for the spray all the links are perfect and we'll get the
37:20 grant then we'll spray the traffic the catch is the holding the traffic and holding the queue I mean the you need a
37:27 big q that means you need debuffer right and also that what we are talking about
37:32 controlling the congestion means look at the traffic on live and if you feel the congestion enable the ecn bits and in
37:40 the end points and using the PFC to do that uh the pause frames and control the
37:46 flow uh we we felt this will be the best options and um uh I we have a lab our
37:56 office and in our uh in our company and we have done kind of a lot of combination of testing and we are seeing
38:03 a good results here that's what I can say and uh yeah we have only seven minutes yeah exactly so these two
38:09 mechanisms the only thing we want to highlight as well is that they can be enabled at the perq level right so based
38:16 on the buffers you have on the switches let's say for the super spine 2 you may have let's say 128 megabytes of buffer
38:23 and then based on that you will decide okay if I'm running my llm model on specific q
38:31 and this llm is very important because it's run I don't know for maybe for some
38:36 governmental agencies or for some financials then I will decide okay to put a little bit more of the buffers and
38:42 then trigger with lower probability all these push backs right so it's important
38:47 to know that it's enabled at the perq level so okay these congestion mechanisms are important but uh it's
38:54 actually better to consider some some of the load balancing efficiency to actually avoid all this congestion
39:00 management because actually congestion management is that the problem occured already and we need to handle this in
39:06 order to avoid the problem of congestion we need an efficient load balancing inside the the backend uh aidc and so we
39:15 have a first example of the pocket spraying where you you see that we have three datagrams and then the first
39:21 datagram of 1500 bytes is splitted inside the switch on six uh cells of 254
39:29 bytes this is quite a typical approach for a uh well the leading uh chip provider on the market of the data
39:36 centers that we split the the the pocket coming on the on the Ingress port to
39:41 multiple cells they are leverag inside the the pipeline of the switch and only
39:47 at the igress buffer they are reassembled and push back pushed on The Wire right so each of these cell gets
39:54 also a a metadata information about the uh uh the the Q IDs as well as the
40:00 lossless uh characteristics right so inside the switch on the pipeline there is some processing that happens but the
40:07 objective of this slide is to say that uh if you have a three datagrams and you run the pocket spraying each of these
40:14 1500 bytes will go on different ports in order to efficiently use the outgoing
40:19 interfaces based on the information of the bandwidth utilization already present on that switch right so if for
40:27 example uh the the port three on the on the switch was highly used by some other
40:32 uh uh llm then in this case only Port one and two would be used in case of of
40:38 the of the bucket spraying right and then we have the second mechanism which is the flow-based uh we may have
40:44 situations where uh uh our Nick card on the server is not tolerating uh the
40:51 reordering of the pockets on the driver itself for specific type of operation of
40:56 the rocky V2 so in this case we may enable for specific operations only uh
41:02 the the flowet mode and then deliver the pockets in order across the fabric so in
41:08 this case with that mechanism of the dynamic load balancing the reordering won't happen right yeah and then the
41:15 last one uh we want to highlight is is the selective load balancing where in fact we decide based on the type of
41:21 operation either read write send receive then we will decide what kind of load
41:27 balancing we'll be using either the flow-based or the per pocket pocket spraying right so this is uh possible as
41:34 well based on some firewall filters and then the next one is a situation where
41:40 we we are not only tracking the bandwidth used locally on the switch but we also actually track the uh the the
41:46 band with utilization and the congestions on the next to next hop right you can see that on the spine for
41:52 example the link X1 in case this one is becoming congested congested then the
41:59 spines will inform the leaf devices that there is a congestion situation and the leaf will incorporate that information
42:05 to decide on which of the outgoing links to send the buckets so some form of uh
42:11 uh traffic engineering will happen but it will happen at the microc level this is the key to remember that comparing to
42:18 the traditional routing uh uh context we are reacting here at the microsc level and not at the at the millisecond or
42:25 second level right so you can see that there are two tables on the switch on the tour one that will be built the the
42:31 local quality table as well as the remote called next to NEX hop quality table will uh incorporate the
42:38 information on the neighbor State and only then the tour device will decide either to send the pockets on ABC links
42:45 or maybe only on the BC to the destination tour four all right so that puff quality is a new thing where we uh
42:52 where we actually track not only the local band utilization but also of of of the neighbor right so this is just just
43:00 a visualization of different load balancing mechanisms you have at the bottom the static load balancing which
43:07 is just H based uh Source desk UDP based this is basic we know that for the last
43:12 uh uh 20 years and then you have the dynamic load balancing and then you have the global load balancing where you
43:18 track your your neighbor links utilization and then you have of course the the DLB where we decide on the type
43:25 of oper ations for which type of DLB will be used I will like at one point we
43:30 have only two minutes to the question but go back to the slide so uh out of this SLB will not work uh because that
43:37 it will it will look for the entropy but it will not for the look for the link utilization and the Q depth so the only
43:43 possibility is the glb or the dynamic load balancing and uh and in the Rocky
43:48 V2 side DC that PFC as ecn that's very important to do the control the
43:54 congestion these are the two very key ingredient to build the lossless fabric
43:59 right Mel and you want to go through that or you any question yeah exactly we'll take the questions just in a in in
44:06 a second so just wanted to highlight at the very end that there is obviously a routing discussion that needs to happen
44:11 right we are all here big fans of the bgp of other routing protocol such as the igps they are also part of the of
44:19 the backend fabric for the aidc so for the front end mahes mentioned at the beginning right it's nothing new we will
44:26 use the underlay overlay in order to deliver the multi-tenancy and then uh monetize the uh the the the deployed
44:34 learning model on the front end but in the back end it's usually deployed as a as just an underlay there's no real uh
44:41 need to consider uh necessarily the the overlays uh one reason is that well we
44:48 we can get a little bit better latencies for these gpus but also that well
44:54 there's a there's there's no no real need to actually enable multiple contexts on the routing side but there
45:01 are other options as well and uh I will talk about this in a second with uh with the igps which of course have different
45:08 characteristics comparing to the to the to the bgp so what kind of bgp is used is the bgp unnumbered RFC 5549 uh that's
45:15 something that is pretty common in case of data center it helps to automate uh
45:21 the deployments so Le to spine will establish their uh bgp perings based on
45:26 the IPv6 link local address addresses being exchanged at the link level and
45:31 then the ASN uh allocations will happen also by either at the automate level or
45:37 or or by the by the Admin itself what I want to highlight is that sometimes these uh networks are connected also to
45:43 the core and then we will be using something called the bgp community for the for the bandwidth so if we have a A
45:51 diversity of the bandwidth when connecting to the core then for the the specific specific destination prefix uh
45:58 the fabric will get actually not only the information about the prefix but also about uh what's the bandwidth uh to
46:05 reach that destination and in this case inside of using uh just IP CMP we'll use
46:12 the unequal cost load balancing when uh when we want to reach the core IP
46:17 networks and then the very last Point uh is that uh bgp may not necessarily have
46:23 the awareness about the topology uh same goes for the link right we talked about
46:28 the community but uh the the at the link level the igps has the native capability
46:35 of of knowing what are the links in the topology what are the links in the specific group of devices right so that
46:42 igp protocol called Rift uh has the the the at the top of the fabric the tough
46:48 level has the um awareness what's the topology look like in the group and how
46:55 to connect to the the other groups to the other PODS of the data center so that's something we also believe is
47:01 important especially if you would like to deploy something called the dragonfly type of topology and so the Incarnation
47:07 of the dragonfly the very simple one you can see on on the diagram here but actually the definitions of dragon flies
47:14 are are really more uh uh more advanced so key takeaways uh so we have uh a lot
47:23 of applications that can be uh uh used in the aidc context different uh large
47:29 language moduls are uh currently evolving so we have large language models small langage models so that
47:35 applications are driving really the deployments dedicated DC infrastructure that the second point with 400 800 Giga
47:42 uh uh increasing thanks to these application deployments and then the last point we covered during this
47:48 session congestion management and the load balancing these are the key two topics uh for the aidc context so thank
47:56 you so much for your attention and uh please if you have any questions we are here uh happy to
48:08 [Music] answer